The data science revolution - Is the CRE industry stuck in the past?
At the 2024 ULI Spring Meeting, Altus expert Sally Johnstone joined panelists Josh Panknin and Drew Conway to discuss the adoption of AI and ML in CRE.
Key highlights
Altus Group expert Sally Johnstone led the ‘Data Science and AI to Answer the Need for Speed in Uncertain Times’ session at this year’s Urban Land Institute (ULI) Spring Meeting
Rather than comparing commercial real estate (CRE) to other industries and verticals, we should consider the gap between what is being done and what could be done if the tools and resources we now have were effectively utilized
The effective incorporation of data and machine learning into your investment process requires a fundamental change in how you think about that process
The AI-driven improvements of current CRE processes are incremental and efficiency-based – they simply allow us to do things a little better than we could before
CRE professionals must learn how to structure and leverage data science tools for our unique problems
The value of any model is directly informed by the value of the inputs and the analysis that goes into the bigger picture
This technology is already here, and it’s only getting better – CRE professionals should focus on the skillset required to combine their talents with available technology to achieve the best results
Machine learning and artificial intelligence can create tools to explain the current state of the industry – and predict the likeliest future
The Urban Land Institute Spring Meeting is a highly-anticipated event that brings together leading industry experts to share their insights on the key trends dominating commercial real estate (CRE) today, as well as those expected to transform the industry tomorrow. This year’s forum gave experts from around the globe an opportunity to delve deep into sustainability, industry forecasts, industrial creativity, and the widespread application of data science and artificial intelligence (AI).
Altus Group expert Sally Johnstone led the ‘Data Science and AI to Answer the Need for Speed in Uncertain Times” session alongside panelists Josh Panknin, Director of Real Estate AI Research and Innovation at Columbia University Industrial Engineering and Operations Research (IEOR), and Drew Conway, Managing Director and Head of Data Science for Two Sigma’s private investment business. Panknin has dedicated much of his career to using practical AI and machine learning (ML) applications to address inefficiencies and create new capabilities within real estate. He also teaches the Artificial Intelligence in Real Estate Executive Education course at Columbia University. Conway leads the research and development of tools designed to directly support the investment process across all of Two Sigma’s private investment businesses, including venture capital, private equity, and real estate.
This session not only provided valuable insights into the transformative impact of AI and data science on the industry at large, but also served as the platform for incredible audience participation through a lively Q&A session.
Is AI being effectively utilized?
According to the US Census Bureau last fall, only 3.8% of all businesses report using AI or data science in their work streams. Comparatively, both McKinsey and Altus Group research has revealed that around 50% of the executives polled reported using AI at some point in their work stream. When asked about their own use of AI, however, over 50% of the ULI audience reported that less than 10% of their work incorporates AI.
According to both Conway and Panknin, this response is not surprising, as the CRE sector has notoriously low AI adoption compared to other sectors. “I can’t remember what historical period came before the Stone Age, but I think we are squarely in that period,” Conway admits.
“To be fair, many other industries still have low adoption as well,” Panknin adds. “Rather than comparing our sector to other industries and verticals, I like to consider the gap between what is being done and what could be done if the tools and resources we now have were effectively utilized. In real estate, specifically, there is so much more that could be done than what’s being pursued right now.”
According to Conway, the question to ask is not necessarily how behind the CRE industry is in its adoption of AI, but rather, why is it behind? “CRE is often a highly personal, in-person, tactile business, where things are changing very rapidly on the ground,” he notes. “At the same time, however, people in this industry have had a lot of success doing things a certain way for a very long time and, with that, can come a resistance to change. To effectively incorporate data and machine learning into your investment process requires a fundamental change in how you think about that process – it’s not that you’re doing something different at the end of the day, but how you get there is very different. I think when people have been successful doing something one way for a long time, they need incentives to make meaningful changes to that process.”
Using data science to unlock better CRE investments
AI and ML are two separate things; AI sits on the automation and efficiency side, while ML analyzes data to harness a deeper understanding of markets and identify patterns. “The AI-driven improvements of current CRE processes are incremental and efficiency-based – they simply allow us to do things a little better than we could before,” Panknin explains. Oftentimes, real estate companies outsource their data science needs, but it’s not always as simple as hiring a data scientist and giving them some data. After all, ML engineers and programmers often have no experience in CRE, and a lot of problems in our industry are not necessarily well suited to AI. With this in mind, we need to ask which problems are best solved with AI – because at the end of the day, AI is not magic. It’s mathematics, and everything has to be quantified.
“ML engineers and data scientists look at things in a very structural way, but they often don’t look at them in a way that represents the nuances, idiosyncrasies and the heterogeneity of real estate,” he adds. “Ultimately, it’s the responsibility of CRE professionals to learn how to structure and leverage these tools for our unique problems, so data science experts that don’t have a background in CRE can build models and tools that fit what we are trying to do.”
Conway notes that he’s seen the most uplift at the intersection of where there is some observable phenomenon in the economy. “When you see things start to repeat in the data, then you can start to form a view around how to approach that community, uncover opportunities for growth, and manage tailwinds,” he shares. “This only happens via a deep integration between a data science and engineering team with investment professionals."
Starting with a relatively narrow view is also a key to success, Conway notes. You need to go deep before you can go broad. “You need the technical understanding as well as the buy-in from the people who will actually be out in the field transacting and sourcing deals. This process requires a deep amount of trust and interaction,” he explains. “We’ve found that when we’re building any kind of AI or ML applications, only about 10% of the process is actually building models. A good 40 or 50% of it is understanding the core business problem, in order to understand what data we need, how to get that data, and how to put that data in the right format.”
AI doesn’t always work – and that’s a good thing
What does it mean when AI fails? According to Panknin, it’s important to reframe the idea of ‘failure’ in this context to an opportunity to learn and build better models. Where the world of CRE and AI technology intersects, we realize that a failure isn’t really a failure. “After a while, you start to see what problems are well suited for AI and what problems are not. There are dozens – if not hundreds – of companies that have been formed to do the same thing, and they fail over and over again for the same reason,” he explains. “Standardization is something that technology does really well. But when you have different information for every single observation, it becomes more difficult.”
For example, extracting information out of a standardized lease document has a high level of accuracy. Extracting information from a lease document that is completely bespoke, however, has a much lower level of accuracy. “If we selectively apply AI to the CRE problems that technology is well suited to solve, we can see a significant gain in efficiency, as well as the analysis and the insights we glean during the decision-making process,” Panknin shares.
Are all data sources created equal?
Given the idiosyncratic and non-standardized nature of commercial real estate data, it’s important to identify which sources of data are better than others. “There is no single source of data that is unequivocally best,” Conway admits. “By understanding the data generation process that each source of data uses, we are able to glean how to benefit and use that kind of variance in our own decision making.”
For example, different data providers will use a different data generation process to provide the market rent estimations for a one-bedroom apartment in your favorite city. Some of them might call landlords and provide a survey, while other providers might plug directly into a leasing system or glean estimations from publicly listed real estate. “There is no magic bullet here, but when you encounter bad data, you have to understand what specifically makes it bad,” Conway adds.
Don’t neglect the bigger picture
One of the things you learn from modeling, Panknin notes, is not to look at the same thing repeatedly. Rather, you need to look at a lot of different things and see how one is going to perform versus the other, given the same or different circumstances. “For example, we once looked at housing price indices for 383 different markets across the US,” he shares. “We noticed that the markets behave very differently – Miami and San Diego had a lot of volatility, while Dallas and Denver were fairly consistent. In this case, we saw different markets behaving differently under the same macroeconomic conditions and political environments, so we knew there was something we weren’t capturing. In this case, we found that it was geographic constraints. Miami and San Diego are in little pockets, so it’s very hard to add new supply when you have changes to demand. The approval process was also much longer in places that had higher volatility.”
In this way, the value of the model is directly informed by the value of the inputs and the analysis that goes into the bigger picture. When you look behind the initial analysis and inputs, what do you find? That’s where the value comes from.
AI isn’t a replacement for human input – it’s an enhancement
As tools like ChatGPT continue to take the world by storm, the question “Will AI take my job?” has permeated across industries.
“This technology speeds processes up immensely, and it makes research really easy as long as you have some domain knowledge,” shares Johnstone. “There is so much upside opportunity to leverage these tools in a meaningful way, you just need to know what your question is, and then craft a model that answers that question. And even if the model doesn’t immediately answer your question, it will likely give you a hint about where to go from there.”
Just as a state-of-the-art race car can’t win a race without its driver, the magic of AI is unlocked by humans, Johnstone notes. This technology is already here, and it’s only getting better. So rather than resisting the inevitable, CRE professionals should focus on the skillset required to combine their talents with available technology to achieve the best results. “Ultimately, AI isn’t going to take your job – but the person who uses it, and uses it well, will,” Johnstone concludes.
Author
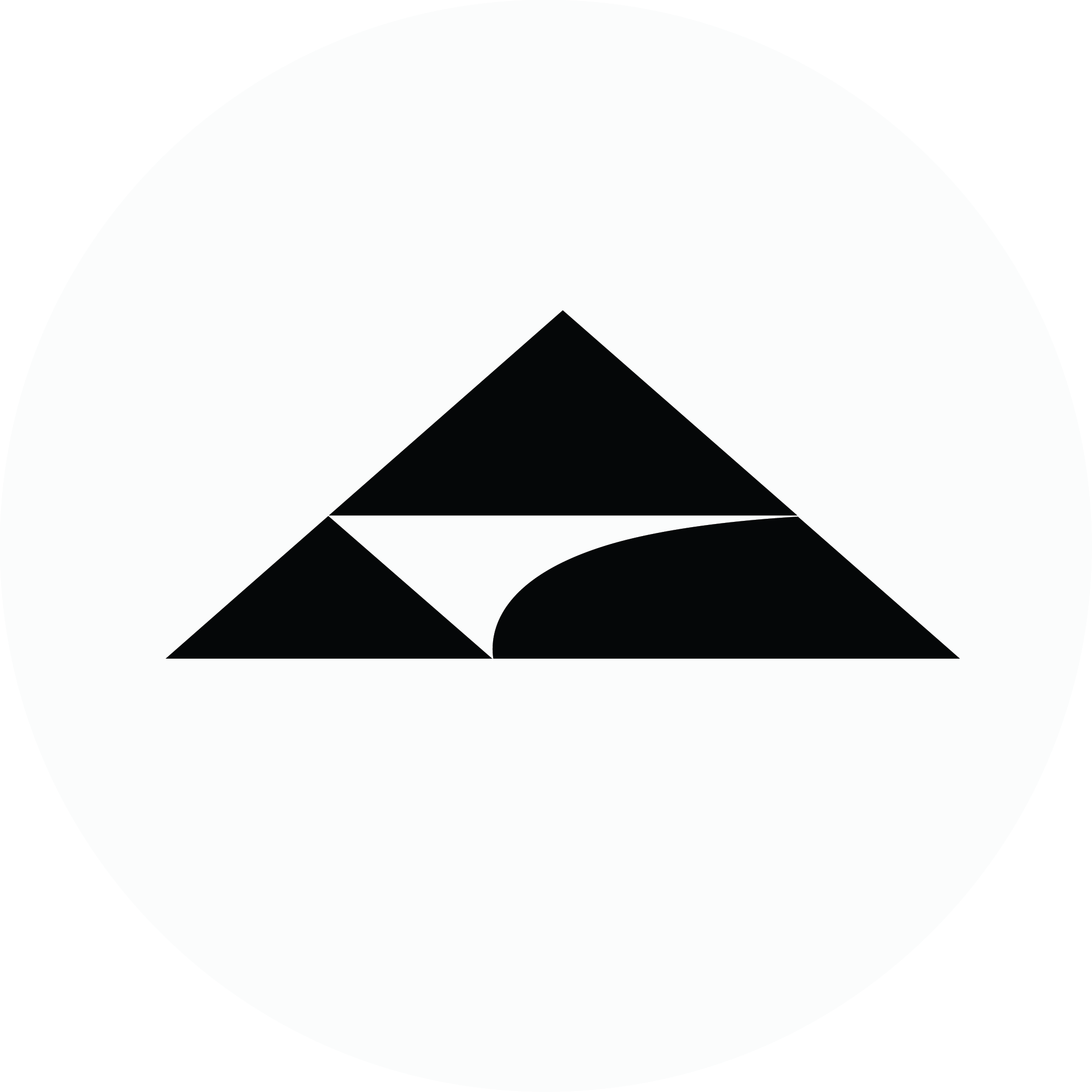
Altus Group
Author
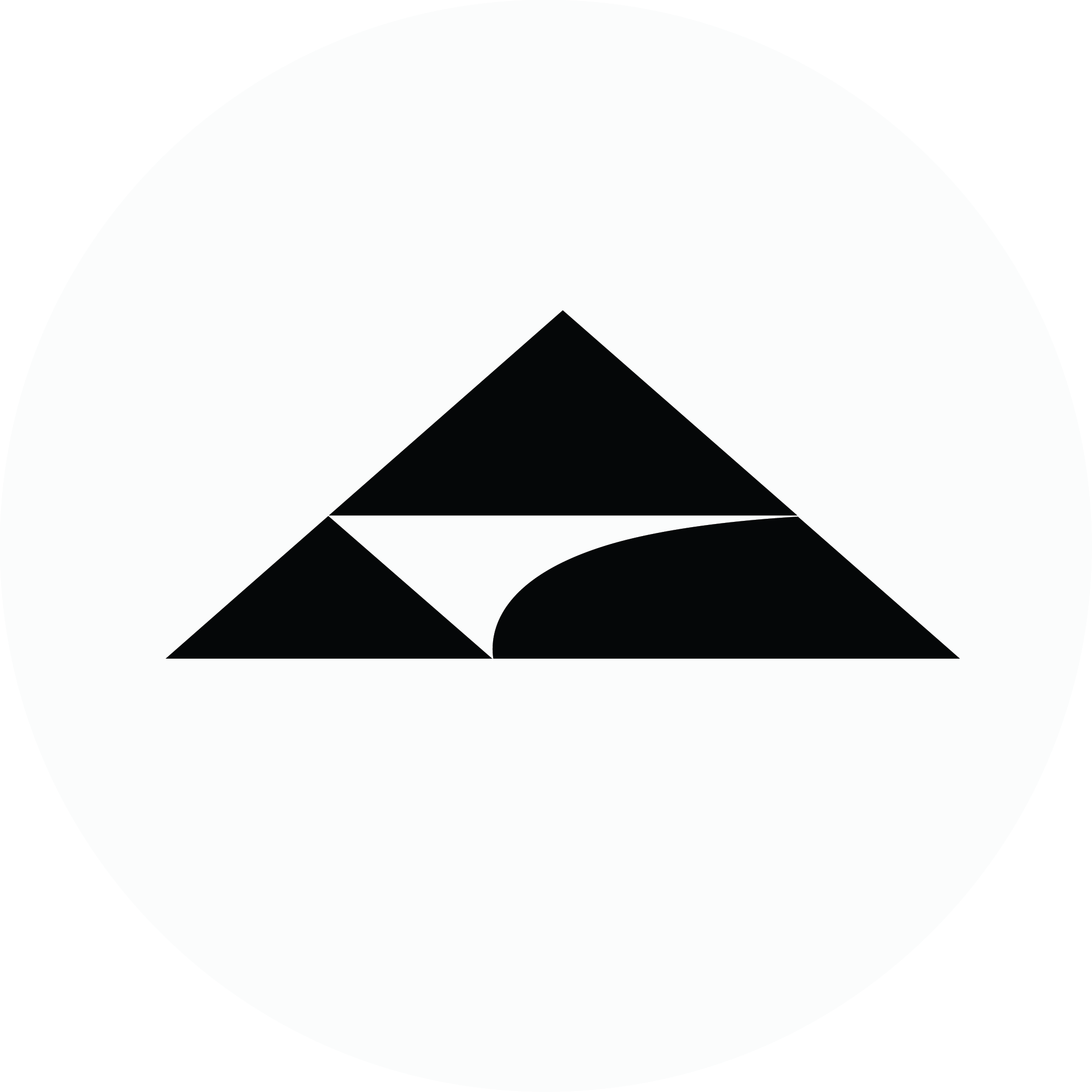
Altus Group
Resources
Recommended insights
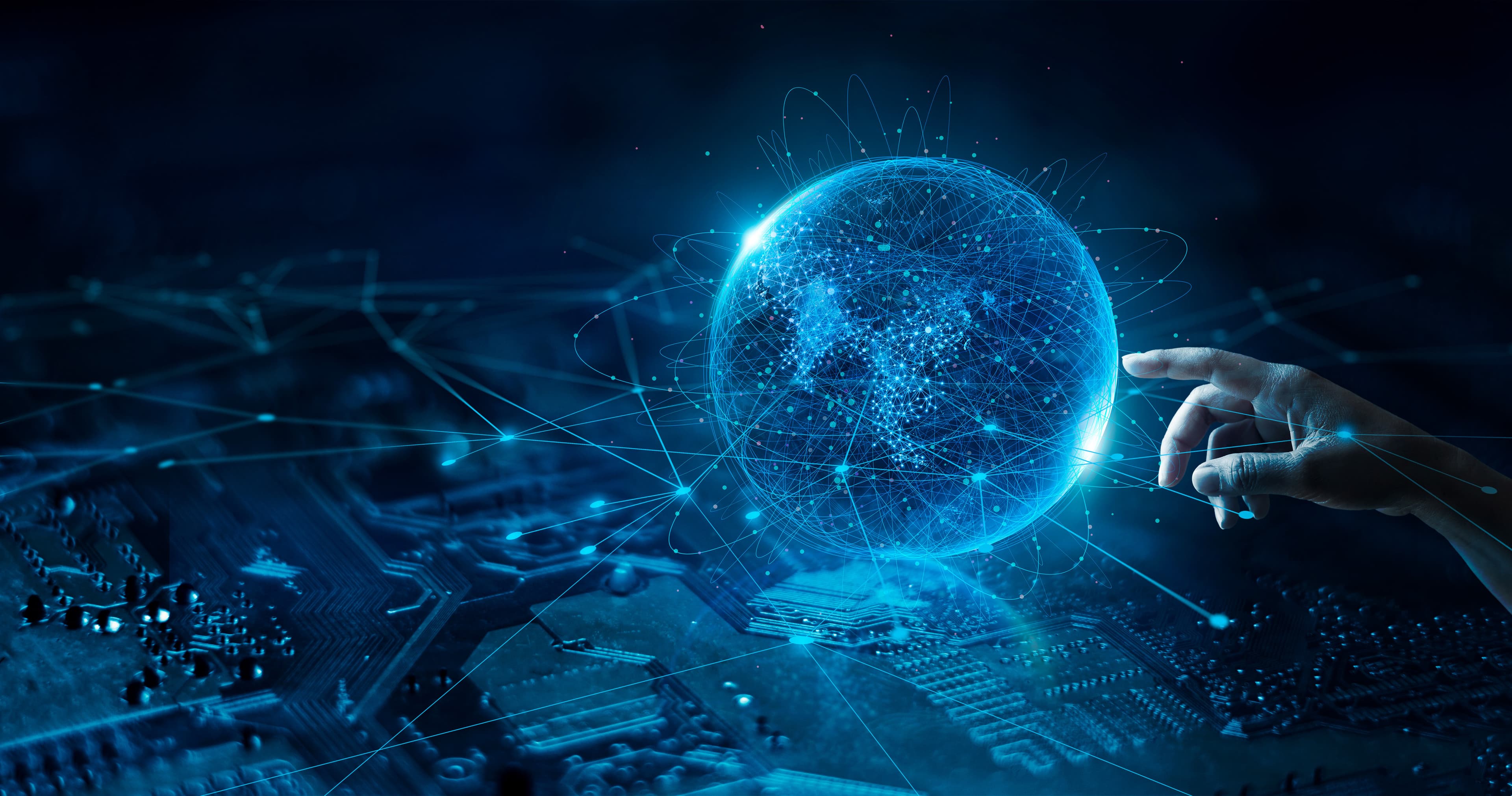
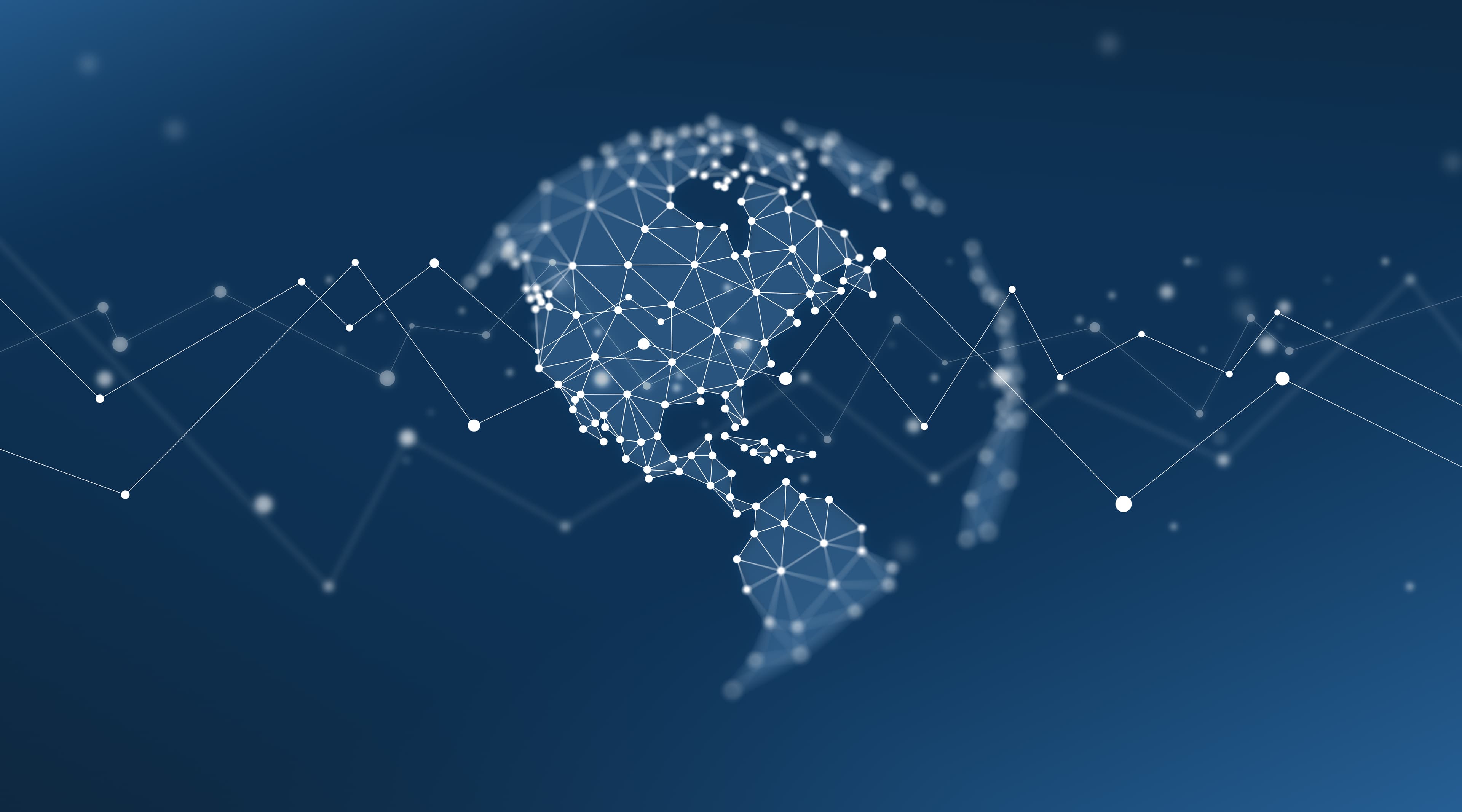
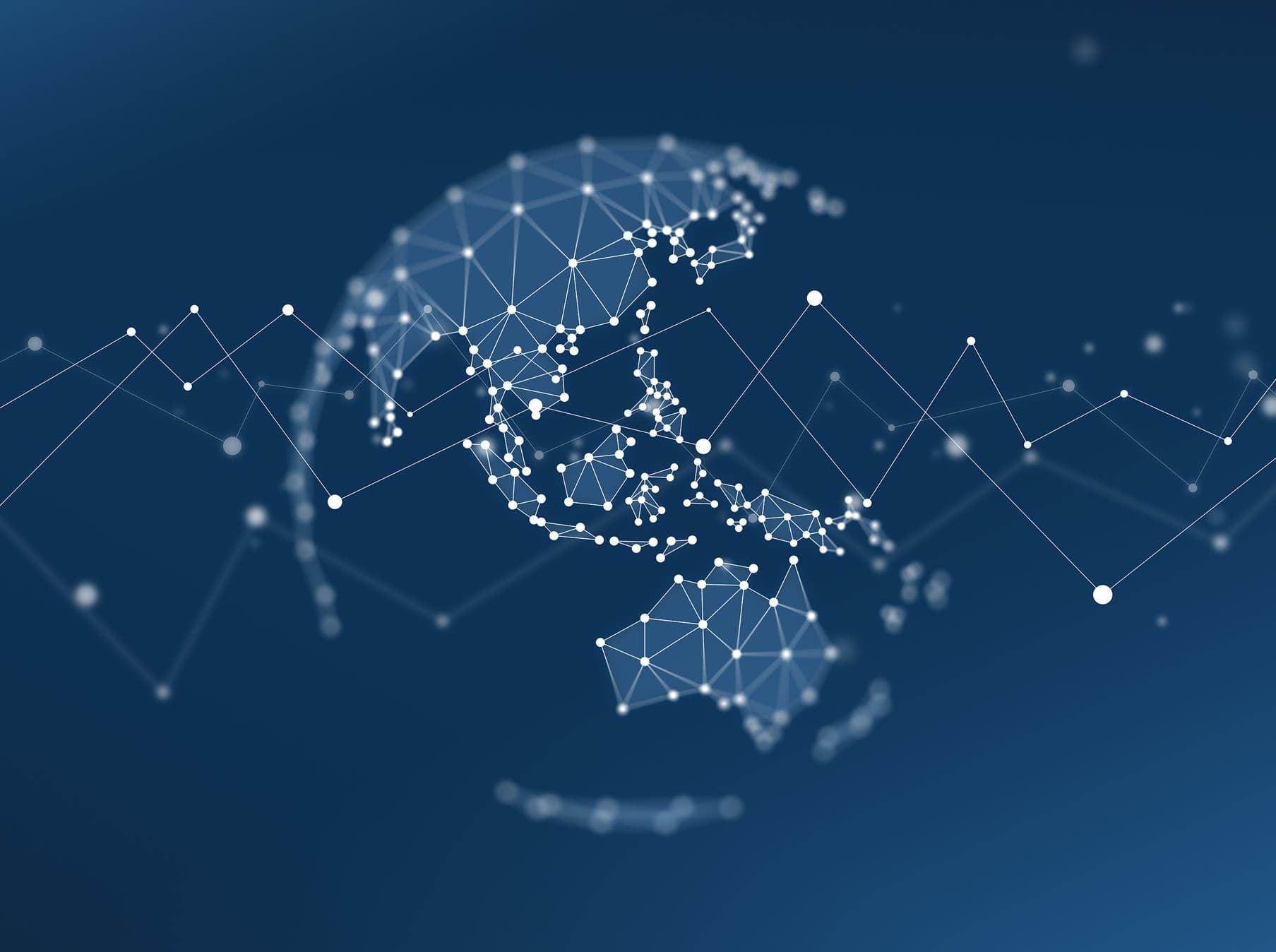
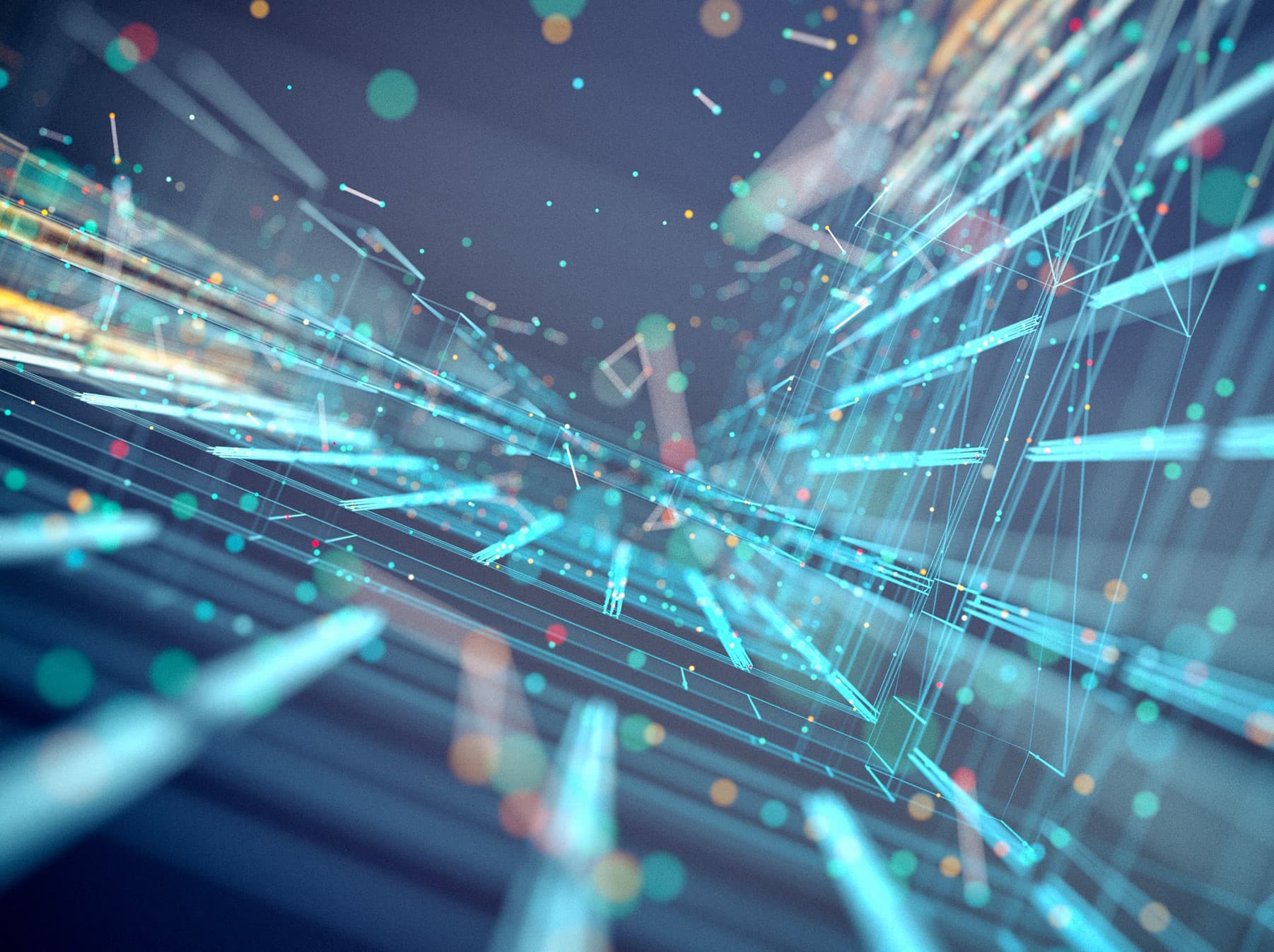
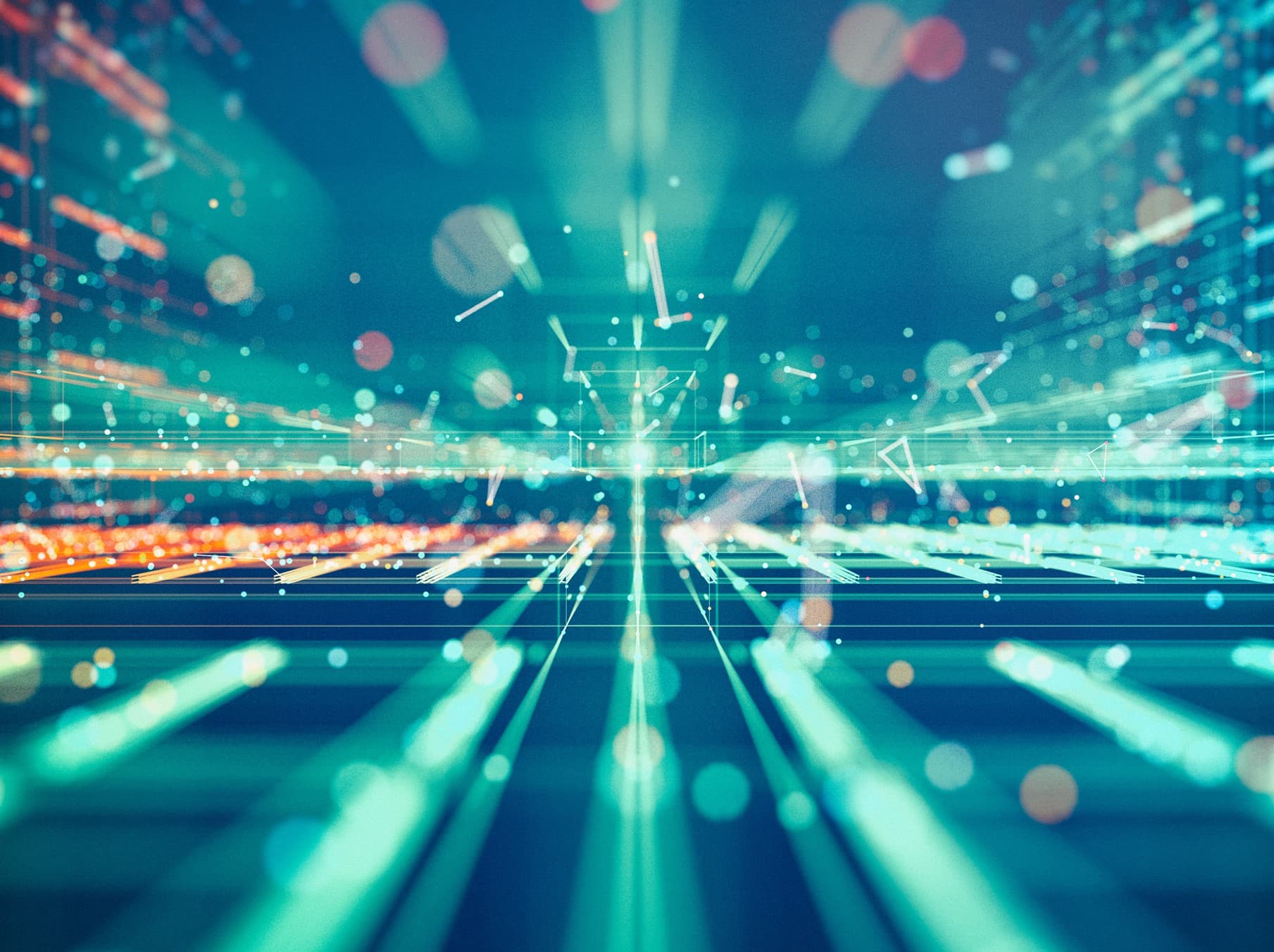