Using advanced analytics and data science can improve decision-making, boost portfolio performance, create operational efficiencies and achieve competitive advantage for commercial real estate firms.
Yet tapping into these benefits can come with development challenges, especially difficulties related to:
Securing internal buy-in for data science initiatives,
Scarcity of quality data,
Hiring or keeping qualified staff,
Uncertainty about the potential return on investment, or
“False starts” or failures where projects did not deliver as expected
Discussing these challenges and potential solutions, a team of our internal data science experts offers a set of key questions and suggestions to help you successfully operationalize data science across your enterprise. These can assist in advancing business goals and delivering a rewarding return on investment.
What do we want data and data science to do for us?
Where do we start?
How do we develop the right data strategy and targets?
How do we gain buy-in and maintain progress?
How do we build a supportive organizational structure?
Should we aim to build capabilities in-house or rely on external providers?
How do we create a data science-driven culture?
1. What do we want data and data science to do for us?
Draw a clear picture of how data can support business goals
“Where data science programs generally fail is poor definition of targets,“ states Michael Clawar, Vice President, Head of Data & Analytics at Altus Group.
“Too often, data science objectives are too general, such as ‘we're going to use data science to drive better investment outcomes.’ You need a clear picture of what you’re trying to solve for – a real business outcome that you want to achieve.”
“The key is to think beyond what data is currently doing for your business and about what data can potentially do to achieve your strategic goals. Determine the business drivers that data can support. ‘Can we offer enhanced returns for our investment strategy?’ Or ‘can we reinforce the market and asset selection process?’”
Consulting with department and unit leads about their challenges and needs related to information and insights helps to gain an understanding of priorities – and also gains buy-in from those whose support is essential for a successful data science undertaking.
Once you have a good understanding of where data can best contribute to your sustainable competitive advantage, you can determine what data is needed to deliver this.
2. Where do we start?
Understand your data assets
Sally Johnstone, Program Manager at Altus Group shares that “taking a data inventory is essential for a clear understanding of your data assets.”
CRE firms collect and use extensive amounts and varieties of data from a range of external and internal sources. Effectively leveraging this asset requires conducting a data inventory. This involves mapping your organization’s data assets and recording:
The type of data you collect
The sources
Where it is held
How it is shared and used
This comprehensive record of information resources helps you understand what data assets you hold, as well as identifying gaps and risks. For example, an inventory can reveal what data you may not be able to capture as part of your current investment process. And related to risks, having an inventory enables you to understand whether you have rights to use certain data and/or whether data includes personal information that may be subject to regulatory compliance.
Mapping also reveals what data is proprietary to you, what specific information you may have that no one else has access to – and contributes to insights regarding how you can leverage this.
Link data science outcomes to business outcomes
Once you understand the data you have, you can prioritize its use based on your business goals and objectives. It’s important to select projects that will return the most value.
“Let’s say you want to increase revenue, for example,” imparts Heidi Learner, Head of Innovation at Altus Group. “Rent tends to be a bigger driver than occupancy levels, so the question might be ‘how can we identify which tenants are at risk of higher turnover?’ Or ‘which leases do we need to negotiate more heavily on renewals?’”
Adds Michael Clawar, “Or looking at profitability: ‘How can we reduce expenses by optimizing tax liability for our portfolio? What data do we have across our organization that can help us understand our tax liability and what it should be? And what data do we need that could help us fill in gaps related to understanding our tax exposure as compared with others in the market?"
“Very specific problems can be tied explicitly to defined ROI. Then the next question becomes, ‘What data sets do we have, or can we acquire, that can help us answer this?’”
3. How do we develop the right data strategy and targets?
Determine what data you need
Once you know what business outcomes you want to achieve, you can determine the data you need to support these outcomes. This can range from market intelligence, economic and demographic information, to real-time data, budget and tax information, property valuations, building operations information, and more.
Now it’s a matter of determining if this data is available and ready for analysis, or whether you need to source it.
Establish how people, technologies, and processes will work together
Consider what key activities need to happen, who will be responsible for these, what changes the business will need to make and the cost to execute. The answers will be the foundation of your formal data strategy, enabling you to identify the projects that will deliver the highest returns.
Designing a reliable data governance framework is also a critical part of the strategy. This comprises the principles and processes that establish how data, people, technologies and processes will work together to achieve goals.
Mitigate the risks of failure
Project risk assessment is an essential component of any new data science undertaking. For example, if you attempt to develop an ambitious model to determine the amount to bid on a property, but you get it wrong, Michael Clawar cautions this could unnecessarily cost tens of millions of dollars for a large acquisition, or potentially more.
“You can prevent a big downside by starting small and failing fast,” he offers. “A quick failure is a win, provided you learn from it. So, think about, ‘Let's assume this goes wrong. How costly is this going to be for our firm if we don't do it right?’ And use this as part of your risk assessment.’”
Examine the characteristics of the data science venture to correlate complexity, cost and probability of success. This form of project risk assessment delivers insight on potential risks and indicates where immediate fixes can be executed to mitigate them.
4. How do we gain buy-in and maintain progress?
Aim for quick wins
Michael Clawar believes that gaining buy-in from end users or an executive sponsor is crucial for these types of programs. He suggests the way to do this is by “having a really clear picture of what you are trying to solve for and then getting quick wins. Very specific problems should be tied explicitly to ROI.”
When launching an initiative like this, aiming for several short-term small wins can help to satisfy the appetite of stakeholders until you’re able to align larger goals with the return on investment.
Benchmark
Benchmarking a data science initiative provides valuable context when meaningful comparisons of performance are tailored to priorities. Well-defined benchmarks can clearly depict the impacts of decisions.
There are different ways to approach performance benchmarking of a data science model or initiative. Benchmarks can measure your current state and compare performance against prior periods or against peers.
Industry benchmarking
“The real estate world is unique in terms of industry benchmarks,” notes Heidi Learner. “For core funds, for example, the benchmark isn't static like those of other equity indices. It is simply the aggregate performance of all funds. So, it’s almost a moving target – you don't know the composition of your industry benchmark until after the fact.”
There's also increasing granularity in industry benchmarks for open-ended funds. At one time there were only a few categories: industrial, multi-family, retail, office and hotel. But with the proliferation of greater investment opportunities, there’s now greater segmentation. So, for example, within multi-family housing, there are student housing and senior living segments.
“This gives rise to the need for more investigation in terms of what it means to ‘outperform,’” says Heidi. “Even if your aim is to outperform your industry benchmark, there are additional questions to ask, such as: ‘Are we looking to beat this benchmark every quarter? Or over trailing periods? is this a risk-adjusted measure of outperformance? What is the time horizon of outperformance?’”
Self-benchmarking
“At the same time, it’s challenging to compare clearly defined outcomes such as ‘we want to optimize renewals around tenants’ or ‘we want to enhance how we're thinking about pricing or concessions that we give to our tenants,’ with industry benchmarks,” adds Michael. “Data is typically proprietary or is not shared and published.” “Instead, it can be more meaningful to establish your own benchmarks: ‘how do our results compare with last quarter? Is the accuracy of our models trending in the right direction?’”
5. How do we build a supportive organizational structure?
Encourage collaboration with hub and spoke framework
For data science initiatives to succeed, research reveals that many companies are utilizing multi-disciplinary “pods” within a “hub and spoke” framework to enable effective cross-functional support.
The hub establishes responsibilities for each pod, operational parameters, and ways of working across the organization. Market or functional “spokes” understand the business challenges and ensure the right questions are asked and answered.
Address the explainability gap
The right working framework is particularly important in commercial real estate organizations since few data scientists have experience in these enterprises – and there can be an “explainability gap” between data science teams and portfolio management teams. How data science models relate to business requirements can be difficult to describe.
This is why it’s helpful to include someone on the data science team who has knowledge of both data science and commercial real estate –¬ an individual who is able to articulate the business requirements to the data scientists.
Broad stakeholder involvement through hub and spoke arrangements also facilitates explaining the data used to build the models and extracting insights from these models. This is particularly important in the real estate sector where the life cycle from acquisition to disposition, and the costs involved, are so much greater than for other equities.
Ensure feasibility
Many models that could work in theory may not work in practice. Involving a range of cross-disciplinary stakeholders from within the organization is crucial to ensure a model is implementable. This broad support facilitates rapid operationalization and comprehensive, pragmatic feedback. Within a few months, people from across the business can identify the data that is available, acquire governance approval to use it, define the problem, begin exploring the data and achieve quick wins.
If you are trying to optimize a lease model, for example, while you may not yet have perfected this model, within a short period of time, you will likely be able to deliver intermediate value, such as an average or a visualization of data, while you continue working on the target data science solution.
Support the initiative financially for the long term
While short wins are critical, data science requires a long view and long-term support. Once key milestones are achieved, enhancing operationalized analytics solutions – those that enable the development of predictive models and conducting analytical tasks – require ongoing support.
Over time, data changes, models shift with new factors and other influences. So, maintenance and improvements require appropriate staffing and budgeting to ensure the data science model is performing optimally.
Michael Clawar points out, “Since it can take years to realize the full value of data science initiatives, patience is a prerequisite, and the view needs to be long term.”
6. Should we aim to build capabilities in-house or rely on external providers?
A variety of factors, including your overall data science goals, organizational structure and culture, influence how you build a team to advance your data science capabilities. Some organizations will choose to build an in-house data science team, others will entirely outsource, and some will rely on a hybrid arrangement. Following are key considerations.
Prerequisites for building in-house capabilities
First, determine whether or not your data science capabilities will be a core competency that's integral to your competitive advantage. “If they are, then it’s not likely you should outsource this,” offers Michael Clawar. “But if your findings are more along the lines of: ‘We need data management support to help us accelerate this project,’ then you might want to consider outsourcing.”
Do you have domain experts within your organization who understand how data influences the decisions they make and how this generates business value? Are they willing and able to help define the framework for a data science project by collaborating with data scientists to solve problems?
Do you have the right tech talent, or can you acquire the right talent to organize and manage the data? Keep in mind that data scientists are among the world’s most sought-after workers.
Do you have management support? Building a team with professionals who have skills that are in high demand does not come without a cost.
Given that the average tenure of a chief data officer is just over two years, it’s also vital to determine whether new tech/data science hires can be successful in your organization. Having an objective third party help to validate potential new hires can be invaluable in this regard.
In situations where the possibility of building strong internal data science capabilities isn’t clear, working with an external provider can reduce risks.
Considerations for external partners
Real estate decisions often rely on securing data from a wide variety of sources, but for many CRE businesses, data is not internally available. Meanwhile, the number of data sources is rapidly expanding while making data compatible with internal systems can be resource-intensive.
This is why CRE firms are increasingly partnering with external providers for a range of data support, from sourcing, to aggregating and cleaning, to managing data sources, analytics and maintaining data governance. Should you decide to consider working with a third-party provider, be sure to take the following precautions.
Quality assurance
To ensure data quality and usefulness when partnering for external data sourcing and management, utilize a quality assurance process to vet third party data. “You shouldn't just assume that data is good,” says Sally Johnstone. Adds Michael Clawar, “Also, some data comes with legal or ethical questions or risks.” In the US real estate space, for example, there are prohibitions under Fair Housing laws from using variables considered to be protected classes, such as age, gender, ancestry, disability, etc. As well, data with personal identifiable information must be carefully secured. Using such data inappropriately can lead to serious financial, regulatory and reputational risks.
Flexibility assessment
If an investment manager wants to access new data sources through an external provider, it’s important that the provider’s data “definitions” align with those of the investment manager. Heidi Learner points to the importance of platform flexibility. “If I’m investor manager that defines my submarket geographies one way, does the third-party provider I’m working with have the ability to ingest my shapefiles so that our submarket definitions match?” she asks.
7. How do we create a data science-driven culture?
Like most major organizational projects, the success of data initiatives relies on the support of people. To ensure that employees embrace collaboration requires investing in data and business literacy.
Employees need to be data literate
Data literacy means ensuring your employees have the right level of proficiency for their particular role and responsibilities. “Data literacy isn’t about transforming everyone into data scientists,” remarks Michael Clawar. “It's about ‘how do we educate other people across the business around ‘what is data? How can I use it? What are reasonable expectations?’”
As a first step in building a data-supportive culture, establish a learning and development program. This should include identifying data literacy goals, assessing employees’ current skills, and assigning appropriate learning paths.
Data scientists need to be business literate
To derive optimal value from data science strategies, the data team needs to be business literate. They must understand the business context of the data they collect and analyze and what value this contributes to the organization.
This requires a business literacy program for new data science hires, which can take a variety of forms depending upon the complexity of your organization and the roles of the new hires. Data professionals’ basic business literacy should include an understanding of these basic components, the big picture: your organization’s vision, mission goals and values
the key measures of success for the organization
how their work impacts the organization’s goals, financial health, and key measures – a line-of-sight from individual performance to business performance.
Some companies develop educational onboarding programs. Others provide continuing professional development. And some arrange secondments where data professionals work in a variety of lines of the business to learn about their challenges.
Both data literacy and business literacy endeavors need to facilitate interactions and relationships among new and existing employees. This helps to integrate all workers into an evolving data-supportive culture.
What’s the downside of ignoring data science as a strategic capability?
What’s the downside of not expanding your firm’s data science capabilities?
Falling behind. Fast.
Data is rapidly becoming invaluable and data science is the foundation of reliable information and insights for informed decisions.
By fielding these seven questions, your organization can address the main obstacles to effective leveraging of your data science potential: alignment with business goals, stakeholder buy-in, data quality, data and business literacy, culture, risks and getting started.
Fundamentally, strengthening your firm’s data science capabilities means doing better. Better forecasting, better investments, better performance, and better financial returns.
Resources to enhance your understanding of data science and its potential for your organization
CREDA (Commercial Real Estate Data Alliance) is a consortium of academics and industry professionals who aim to improve access to and understanding of available data in commercial real estate to foster higher quality research and interactions between academia and industry, to the benefit of the entire CRE community.
ODSC (Open Data Science Conference) brings together the global data science community to encourage the exchange of innovative ideas and the growth of open-source software.
NABE (National Association of Business Economists) Tech Economics Conference is an intellectual exchange of leading thinkers in economics and data science regarding how interdisciplinary developments are impacting today’s approaches, frameworks, tools, and techniques.
Author
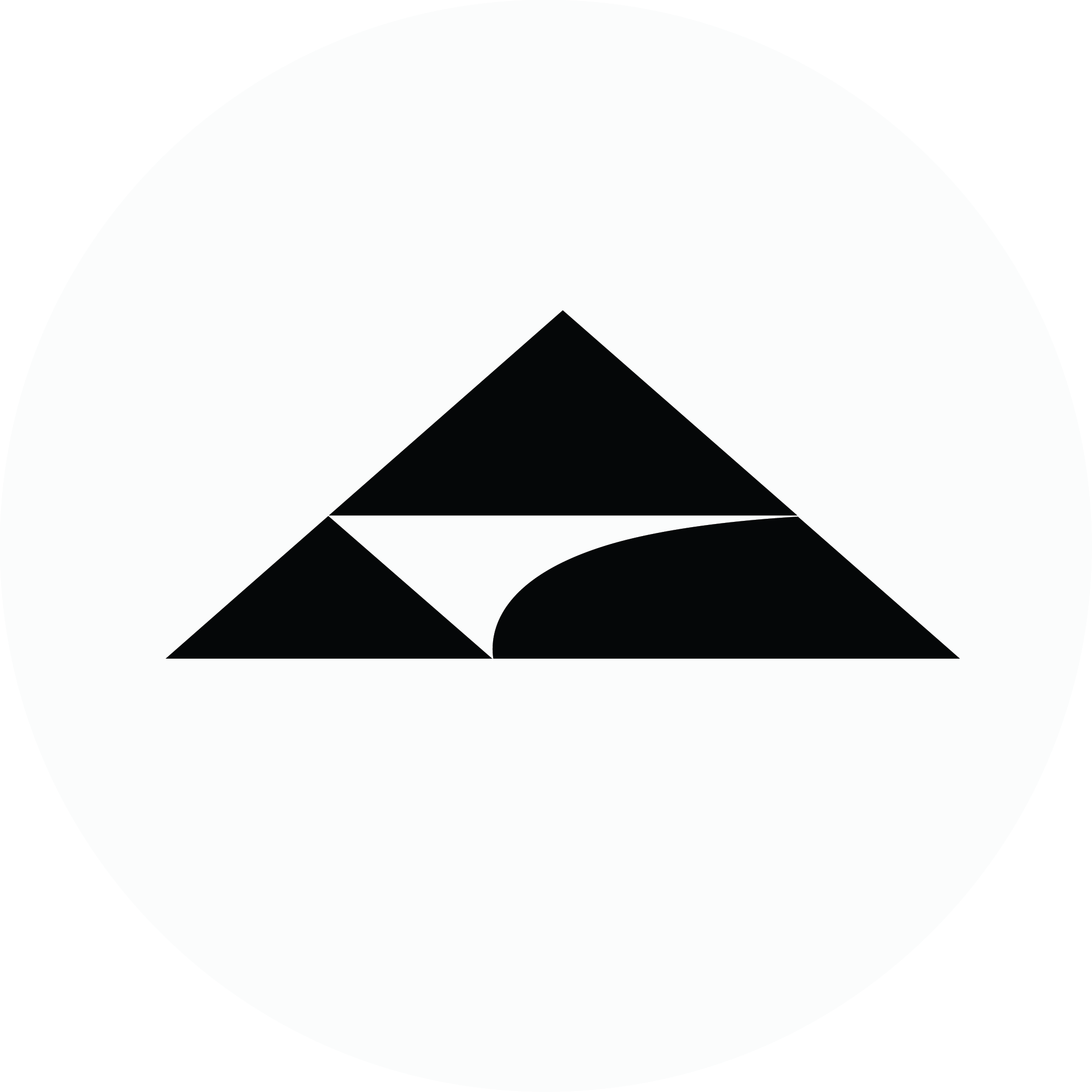
Altus Group
Author
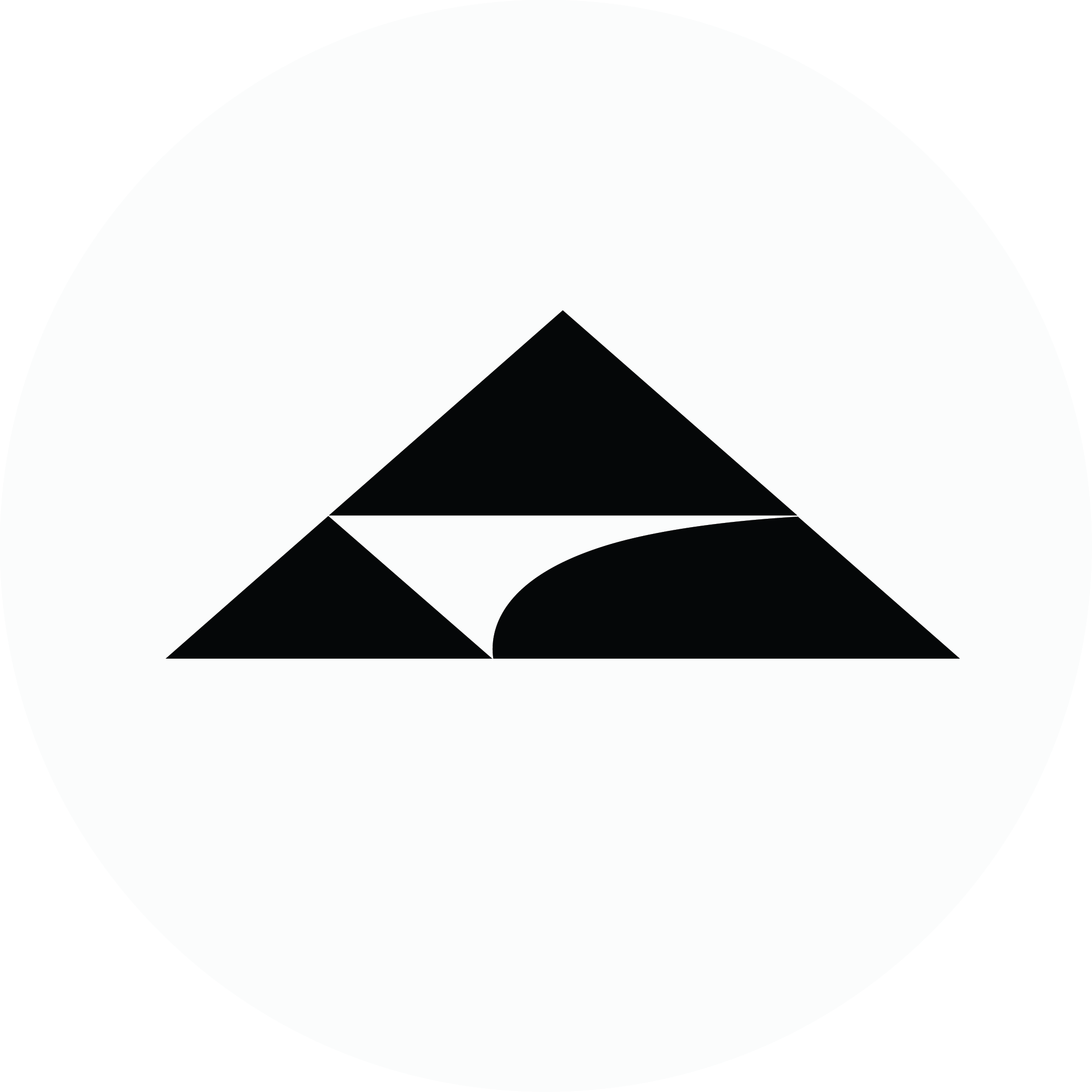
Altus Group
Resources
Latest insights
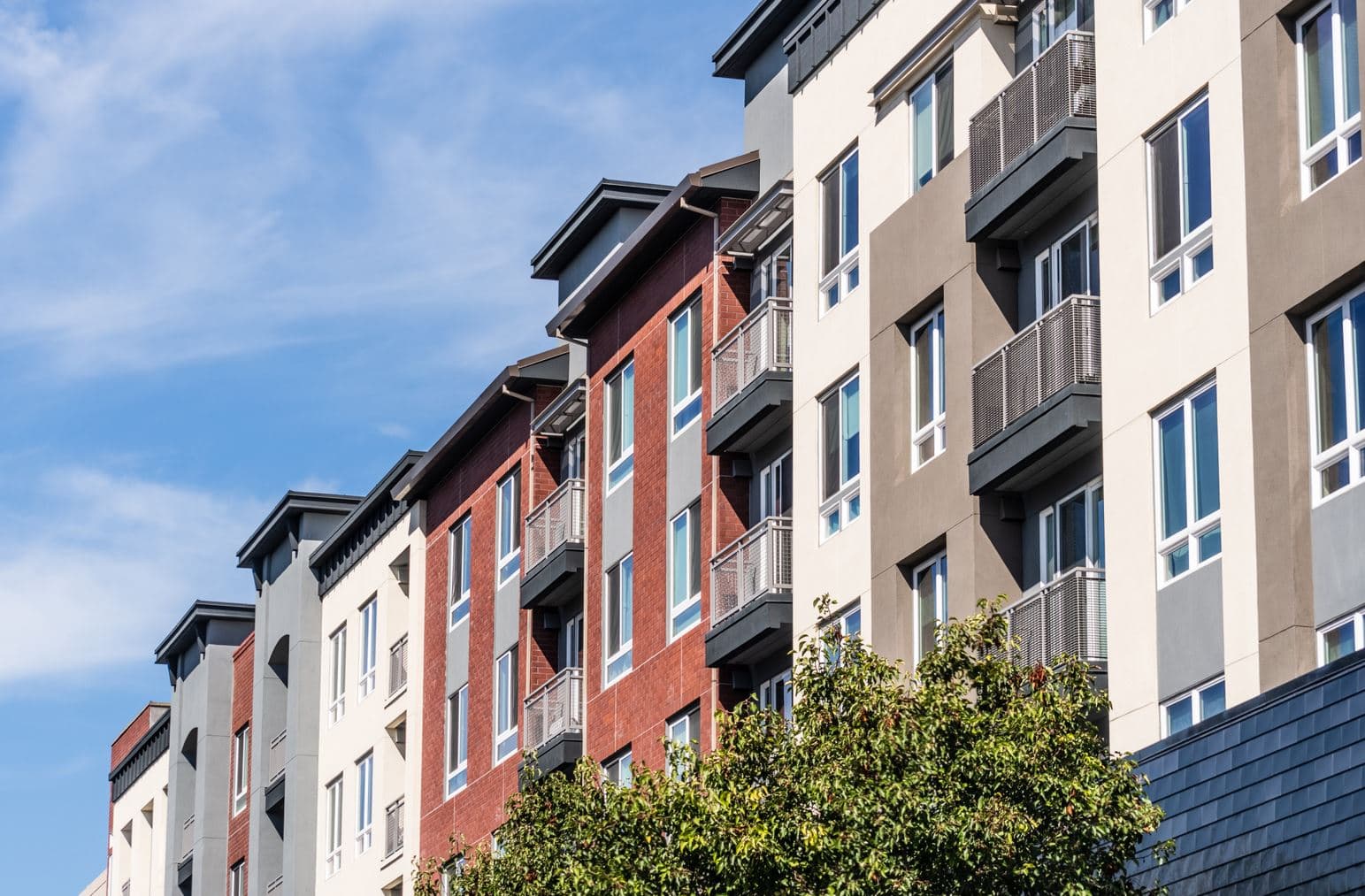
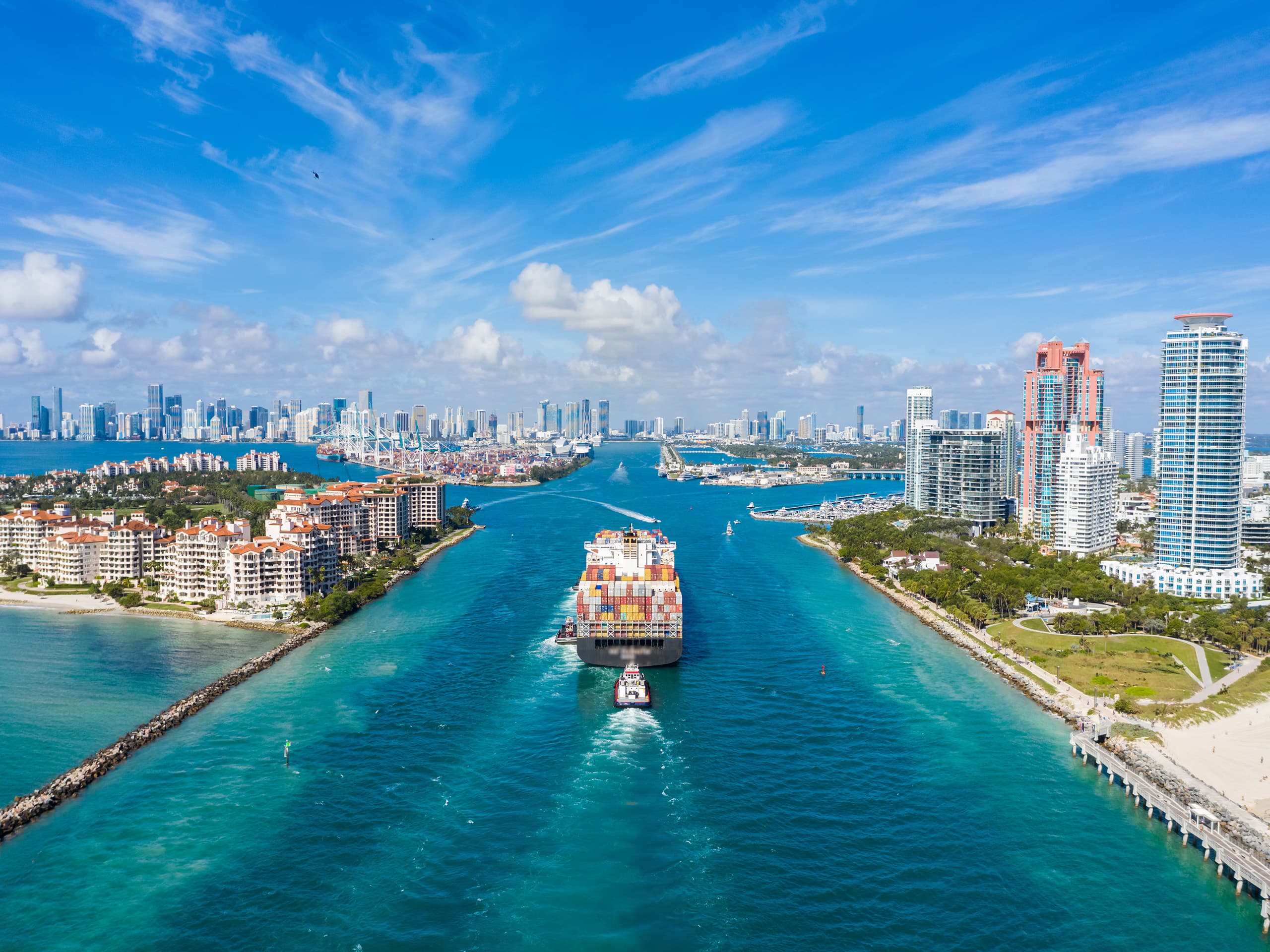
Sep 12, 2024
The 5 US cities for industrial investments that are most likely to outperform benchmarks
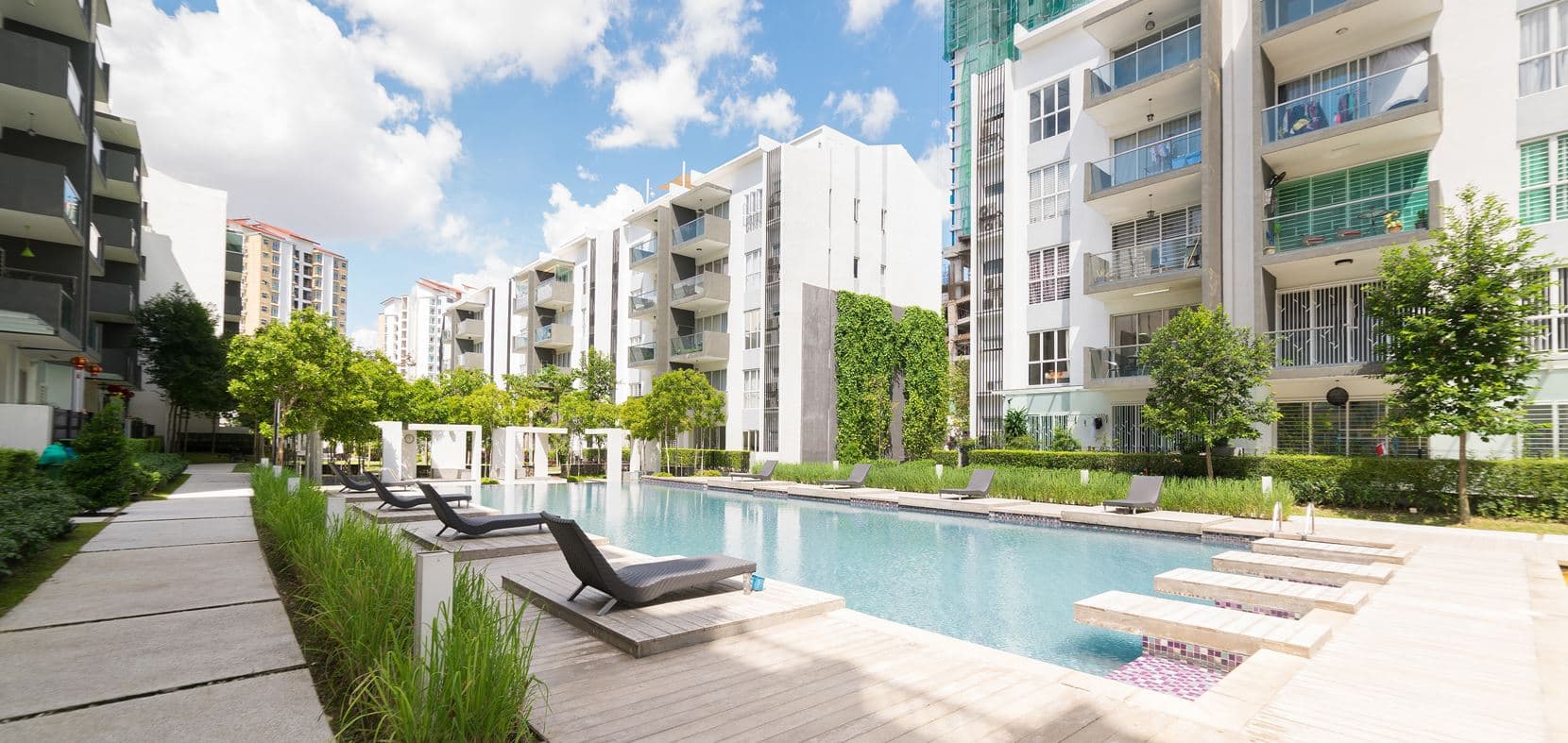
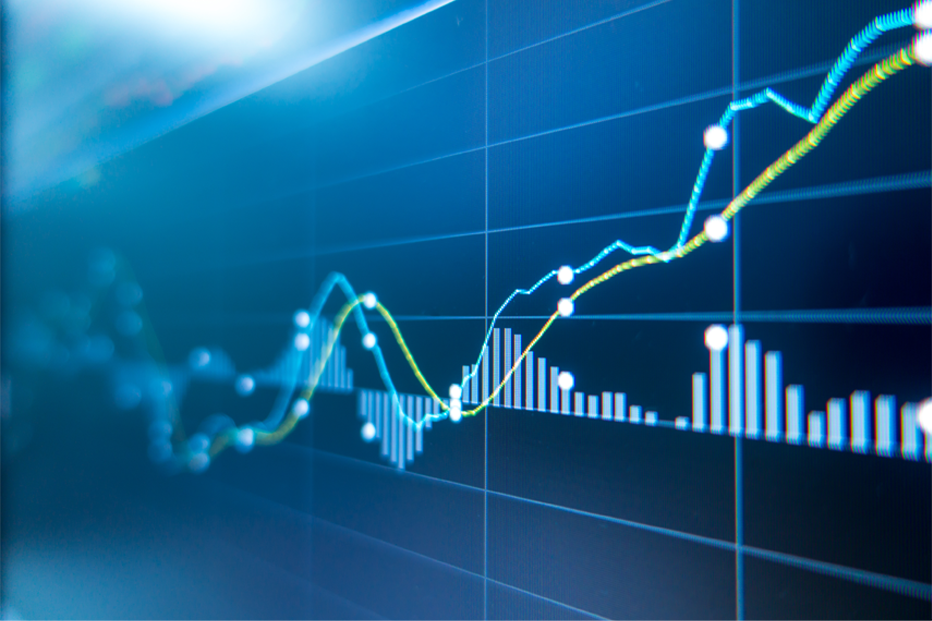
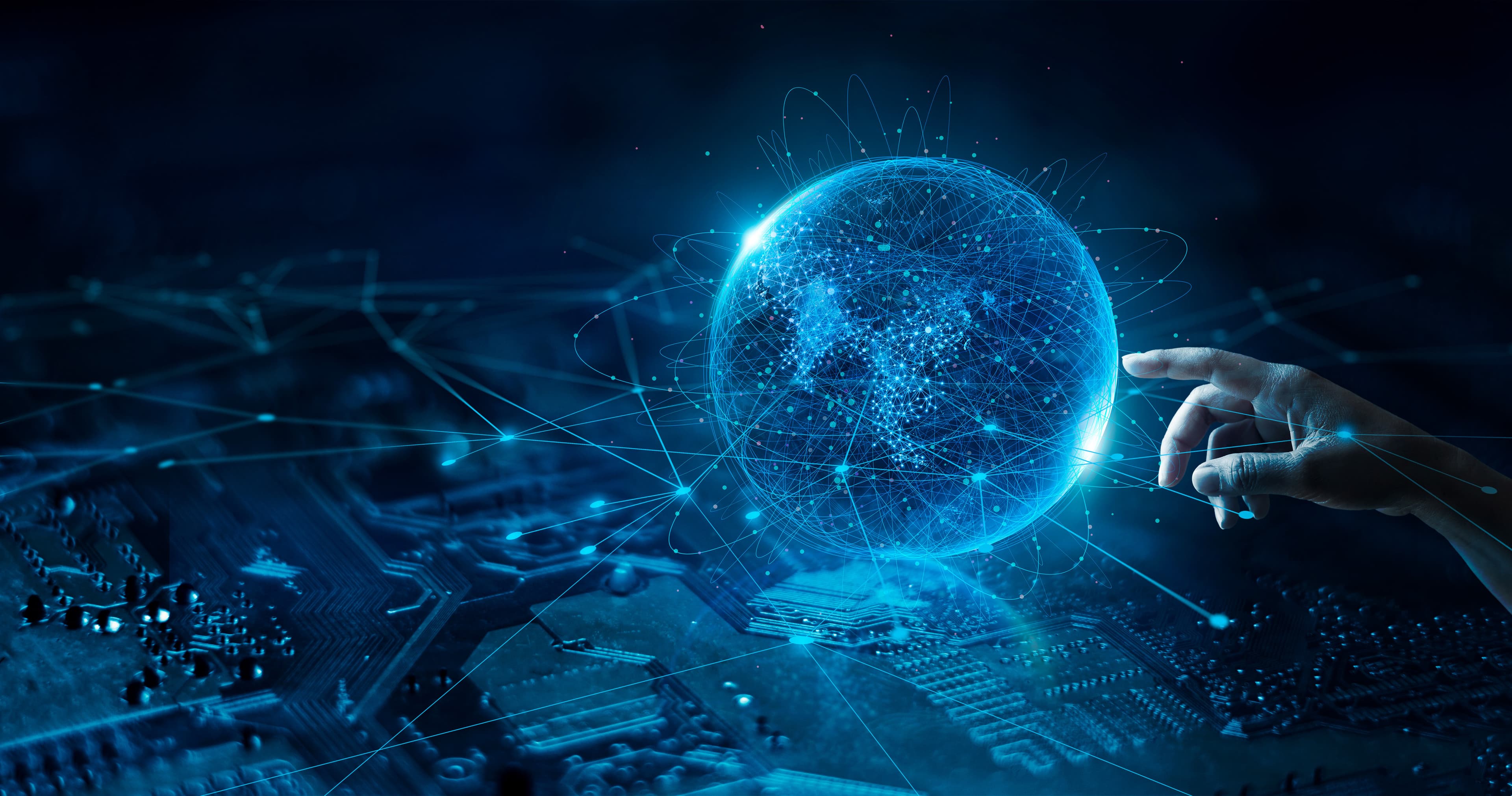