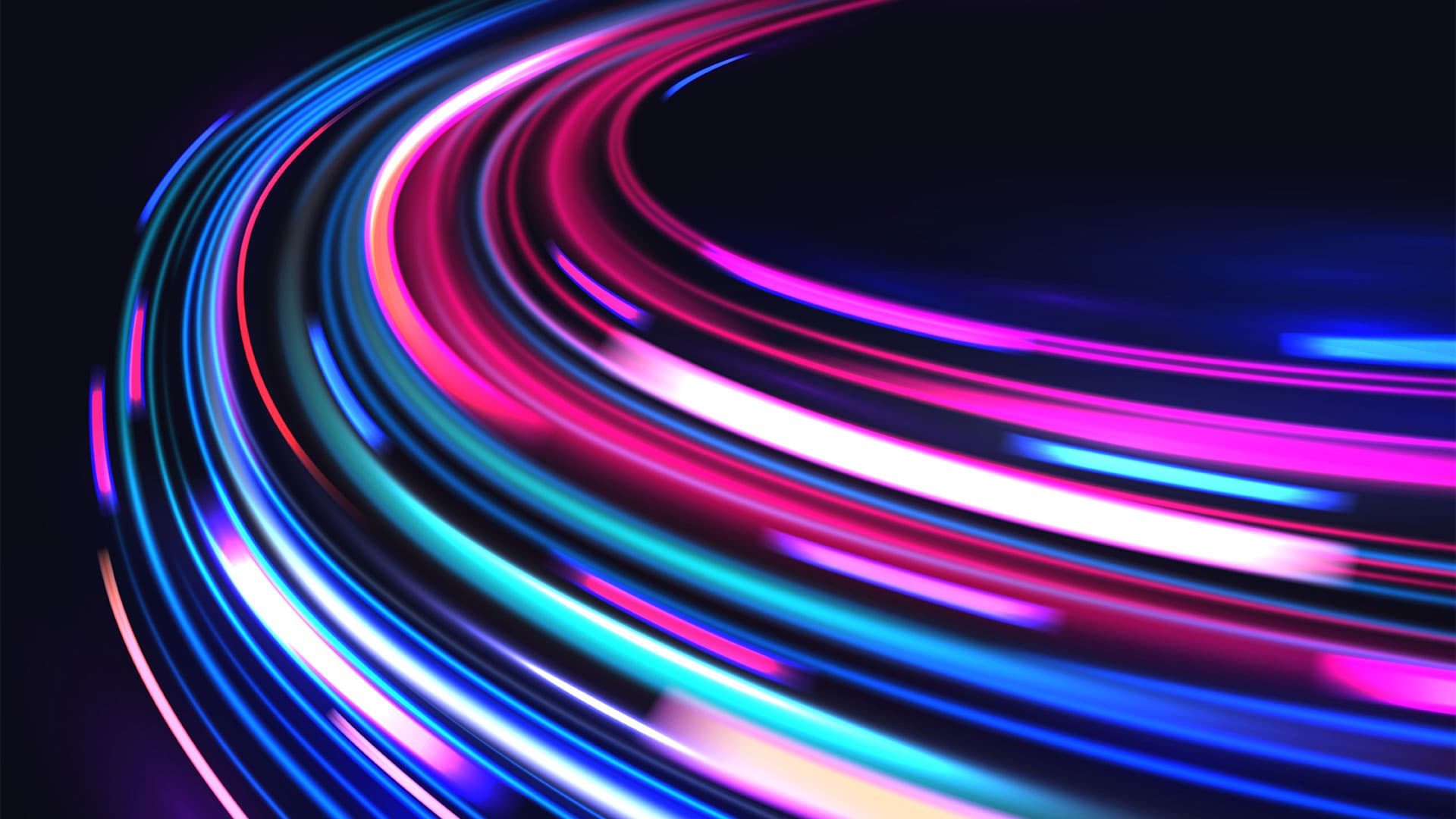
EP3 - From ideas to impact - Building tomorrow's proptech
Explore how proptech is reshaping commercial real estate with tools that deliver real ROI, streamline operations, and enable a more connected future.
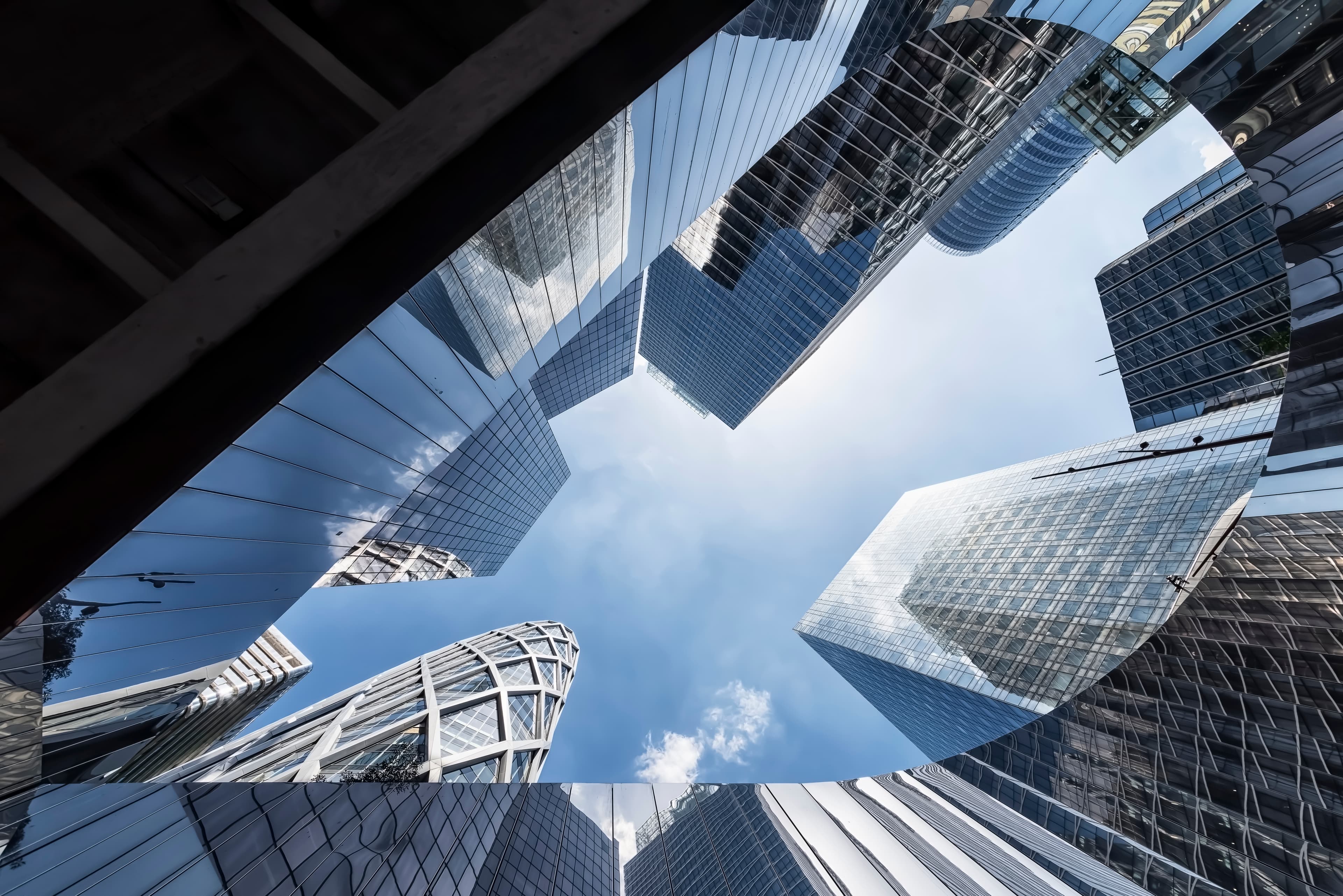
Scaling smart in European CRE with valuation management outsourcing
As European CRE markets rebound, learn how outsourcing valuation management helps funds scale quickly, stay compliant, and refocus internal teams on high-value priorities.
What a quarter century of transaction data tells us about US commercial real estate
We examine the long-term trends that have reshaped the commercial real estate landscape and what they may suggest about its future.
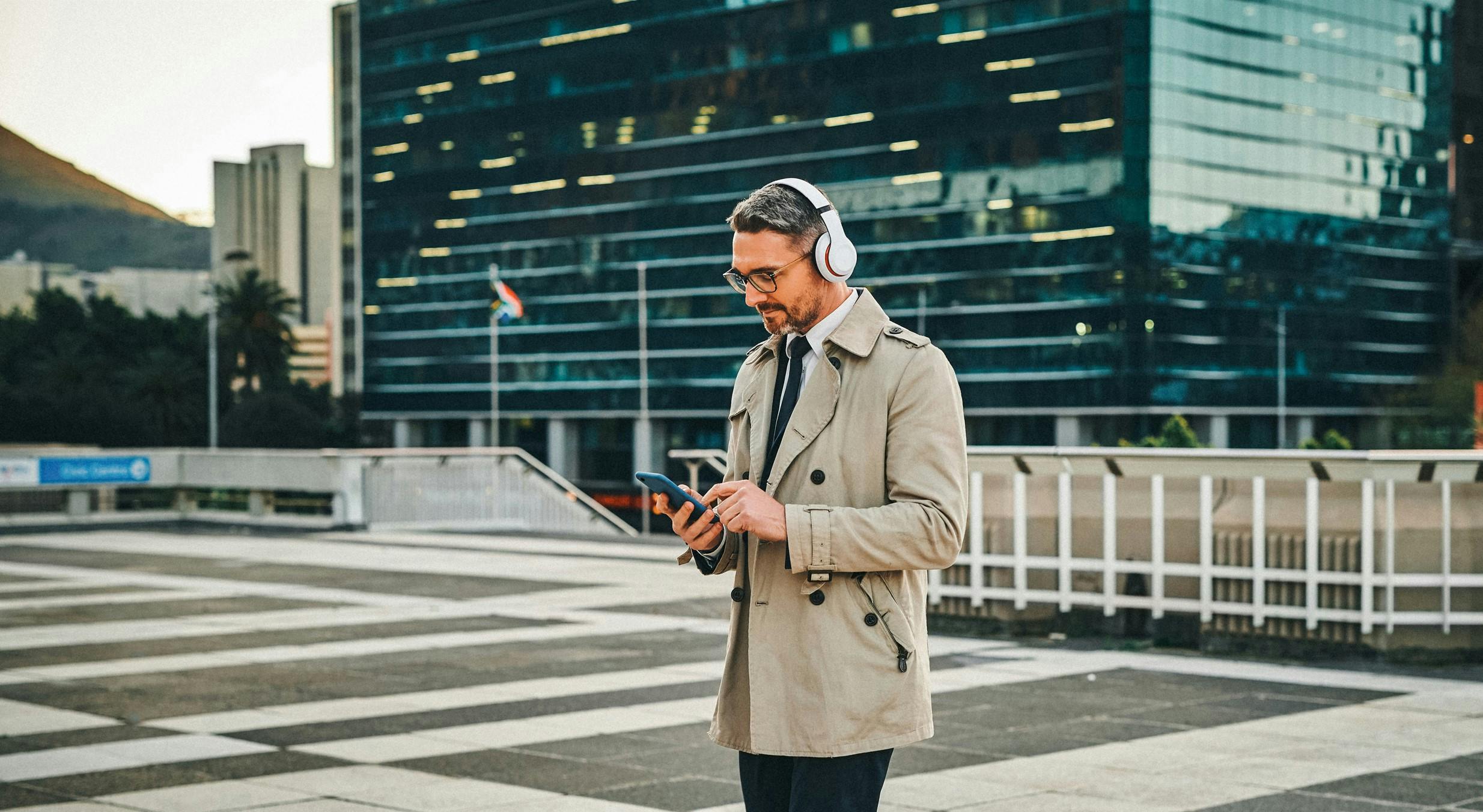
EP67 - CRE’s mid-year market pulse: Rates, risks, and real assets
Catch up on key trends in CRE as we assess economic signals, capital access, and the growing role of real assets heading into the second half of the year.
Featured
Insight
Research
Podcast
Resources
Latest insights
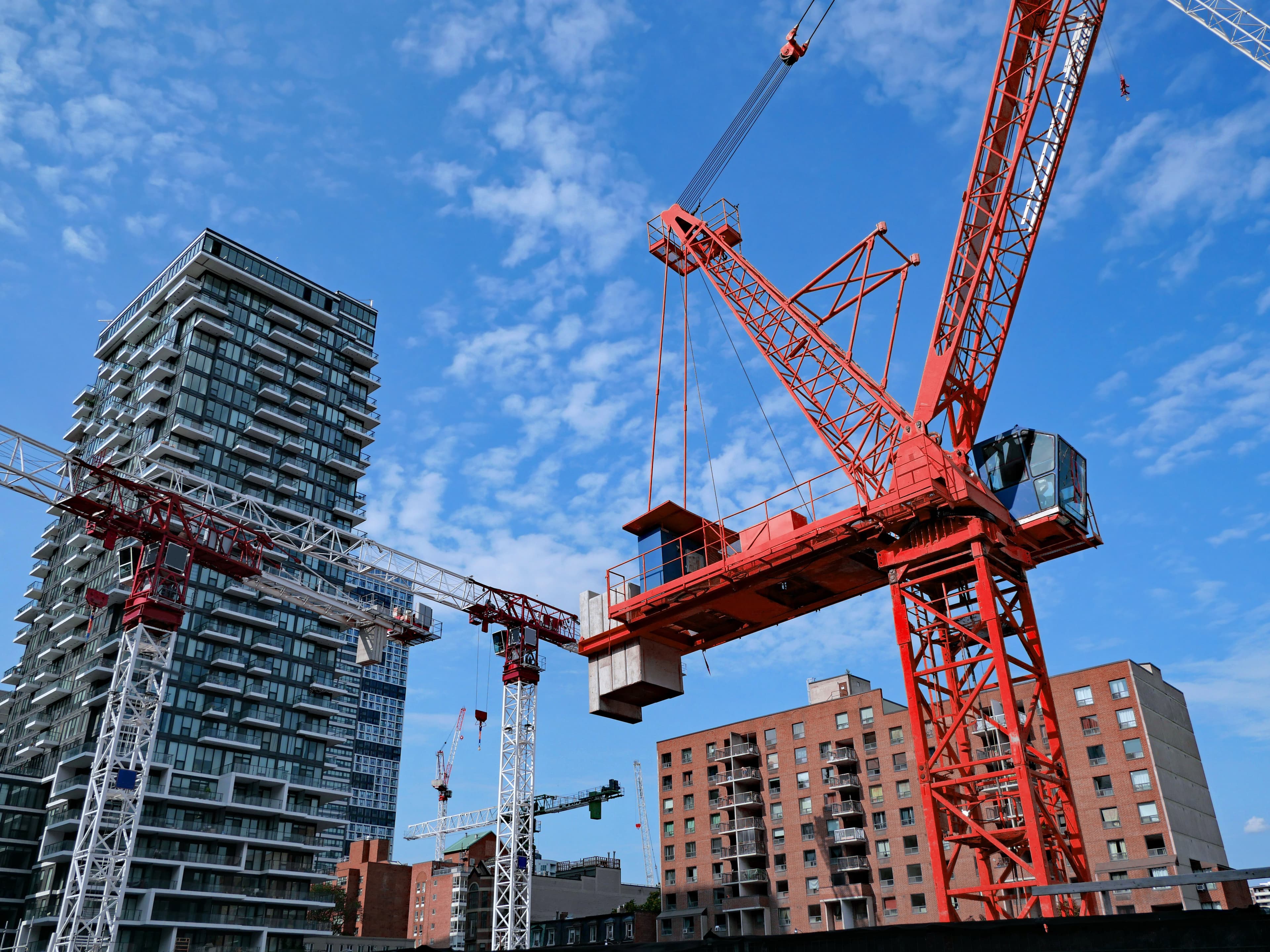
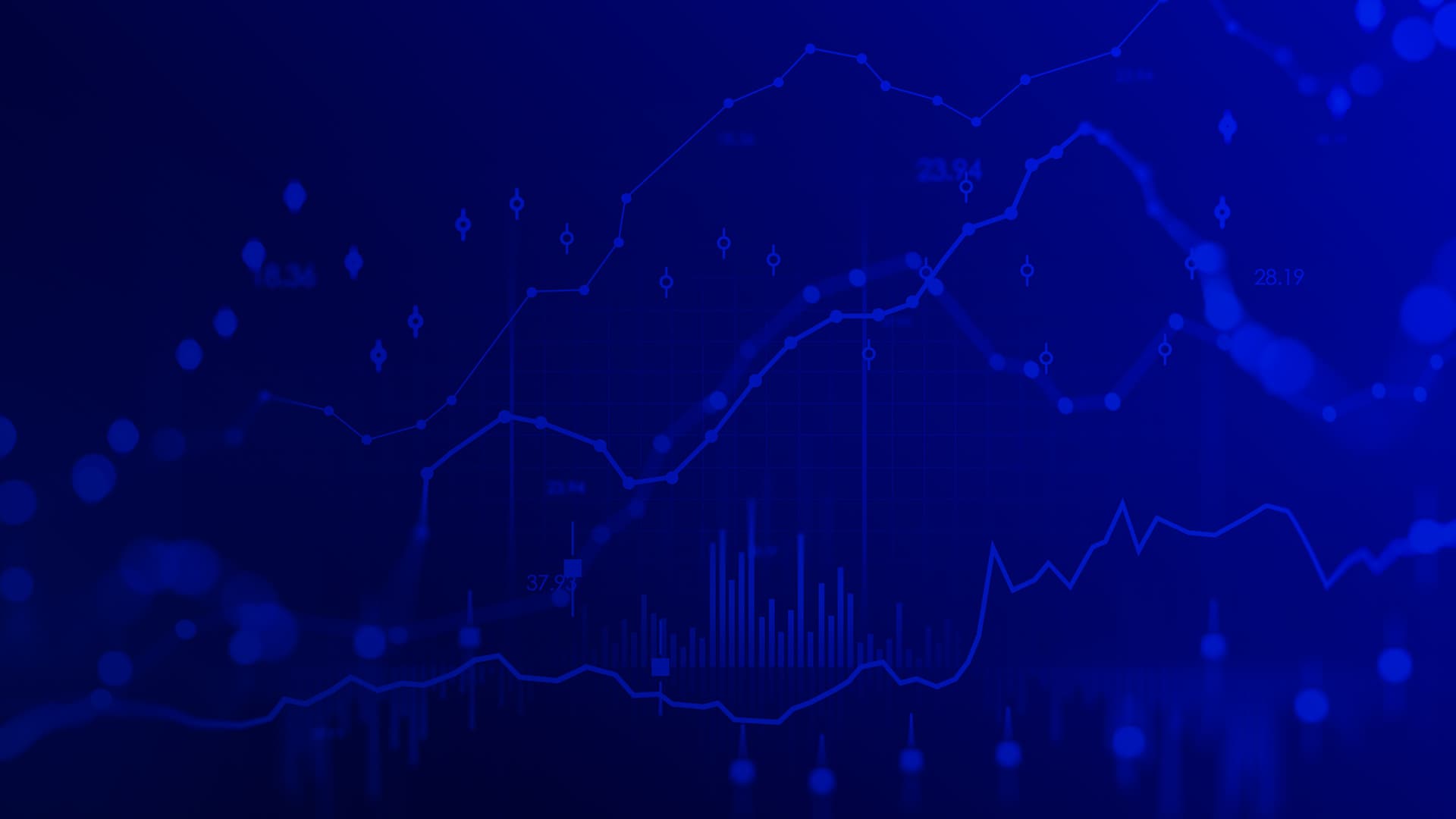
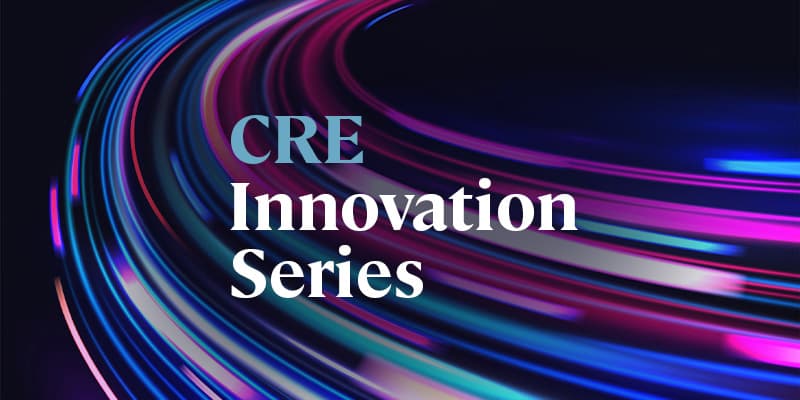
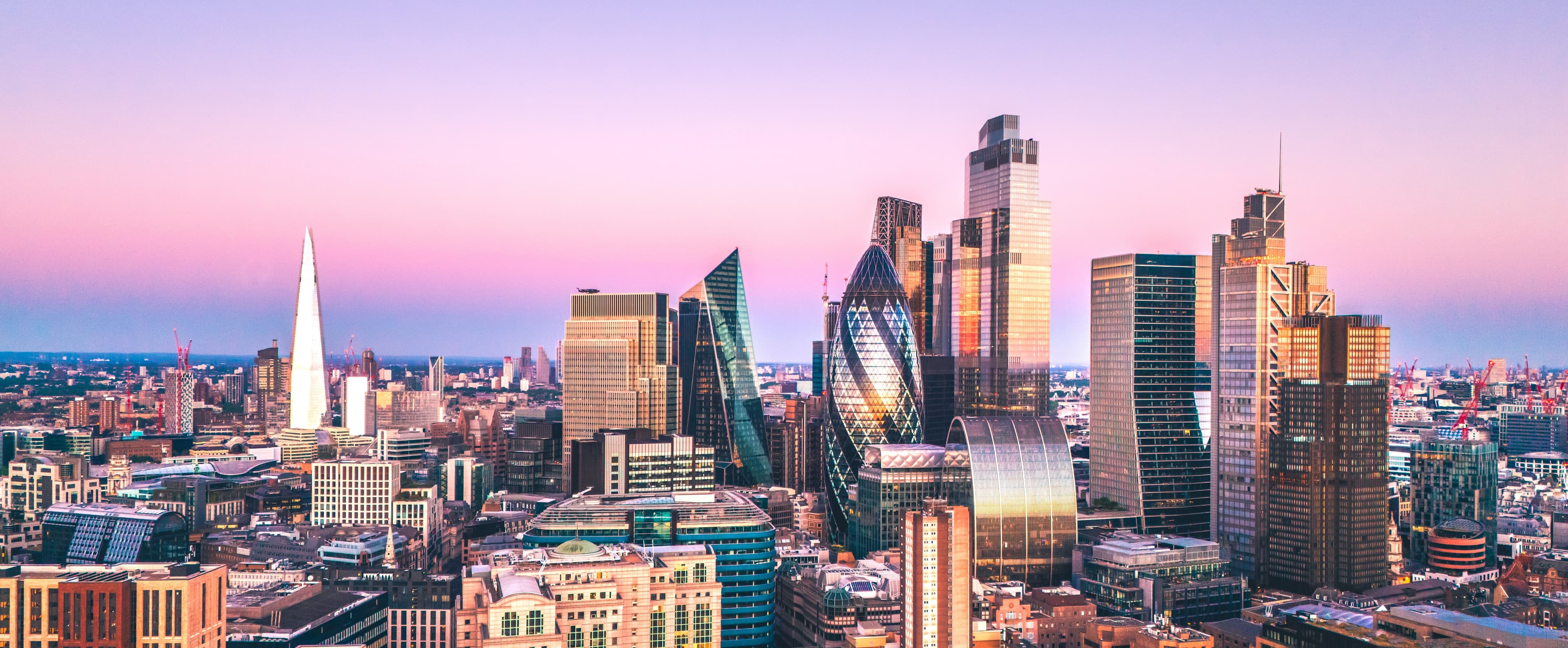
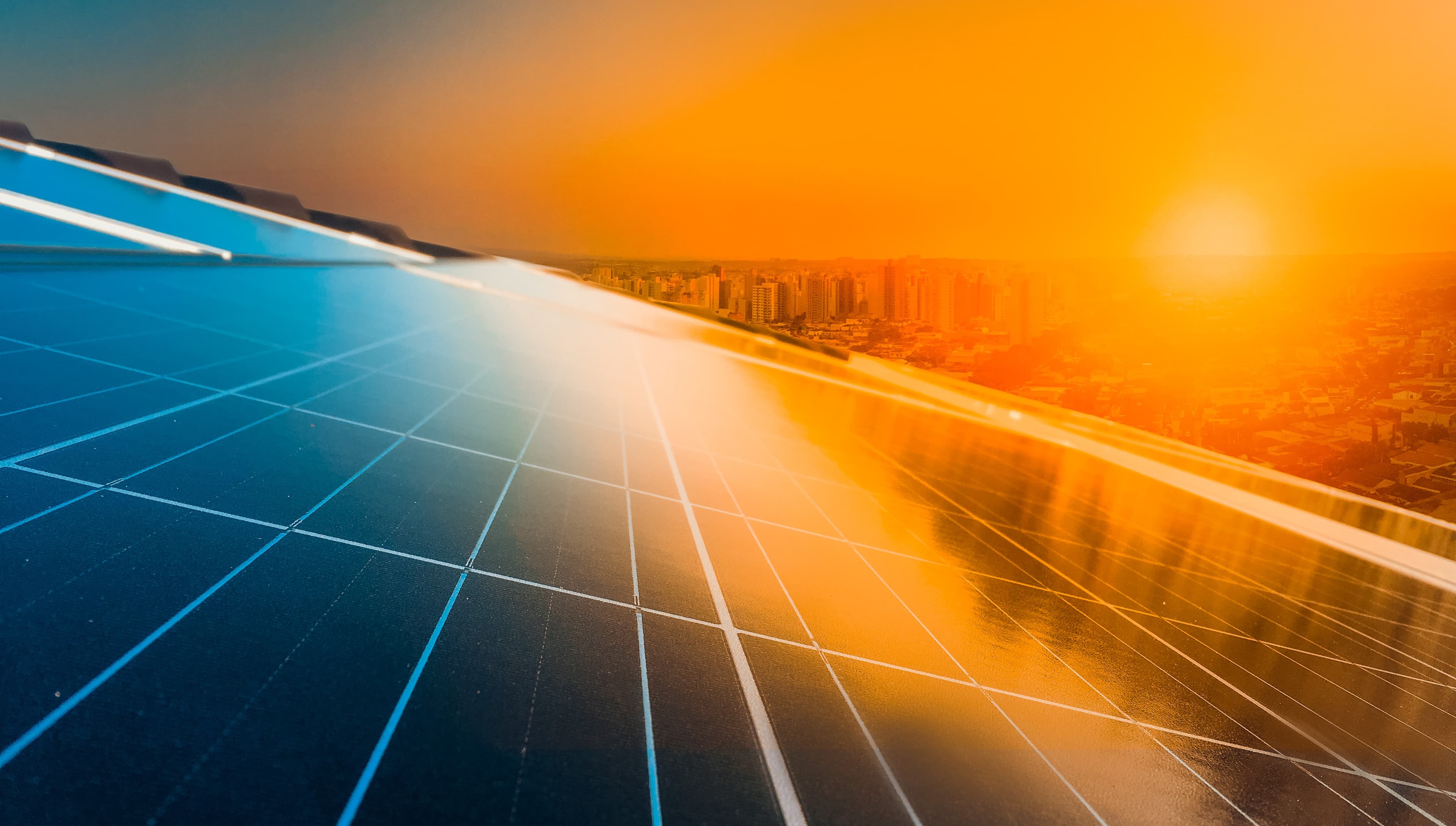
Jun 24, 2025
The path to properly reflecting decarbonization efforts in Canadian CRE valuations
Trending articles
Featured insights
Australian construction price outlook - Q1 2025
Combining our market intelligence with robust data sources to provide the Q1 2025 quarterly Australian construction material price indicators for the construction sector.
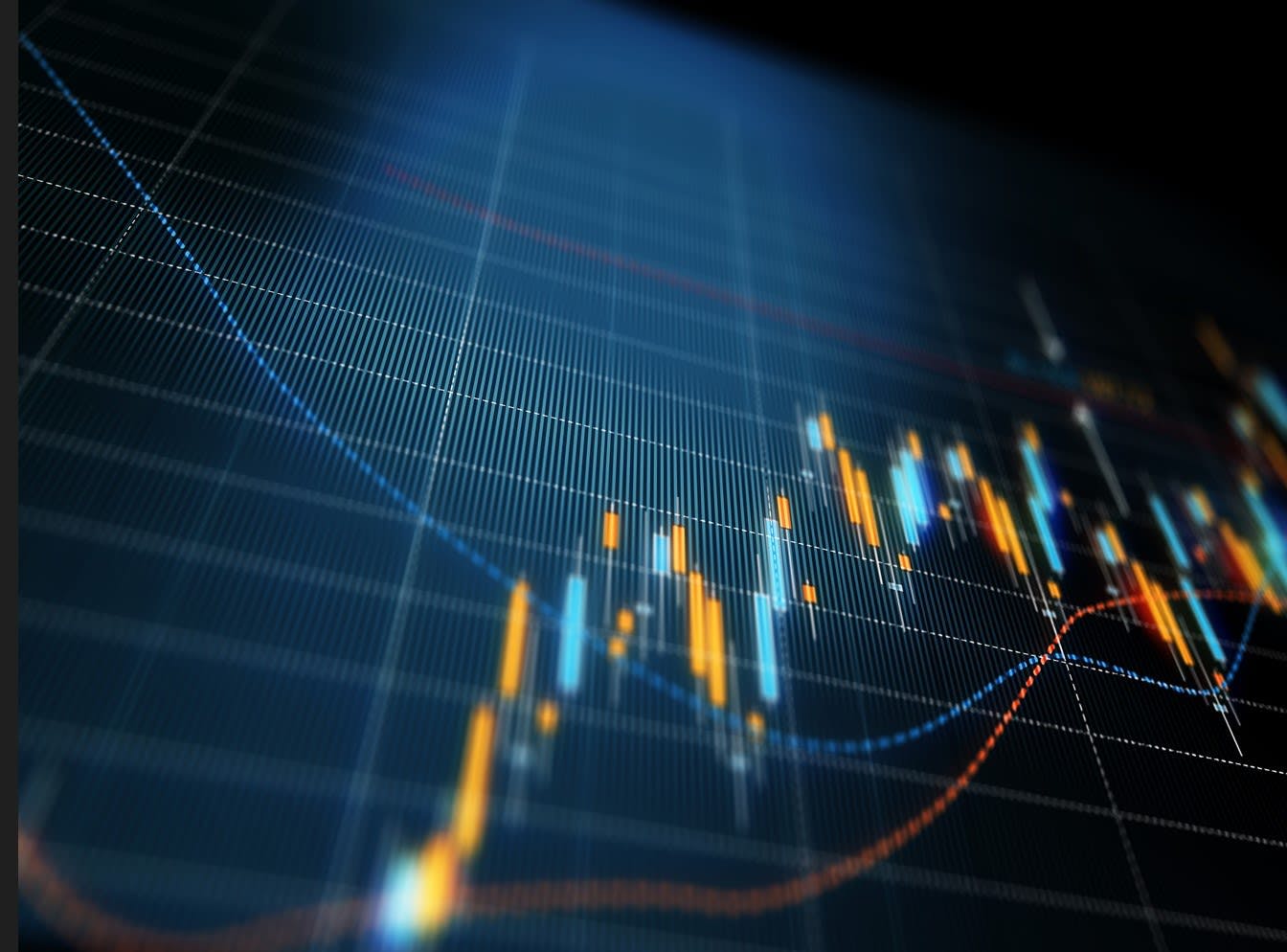
Featured insights
Canadian commercial real estate market update - Q1 2025
In our quarterly update on Canada's commercial real estate market, we discuss investment trends in Canada's five major markets: Toronto, Montreal, Vancouver, Ottawa and the Greater Golden Horseshoe.
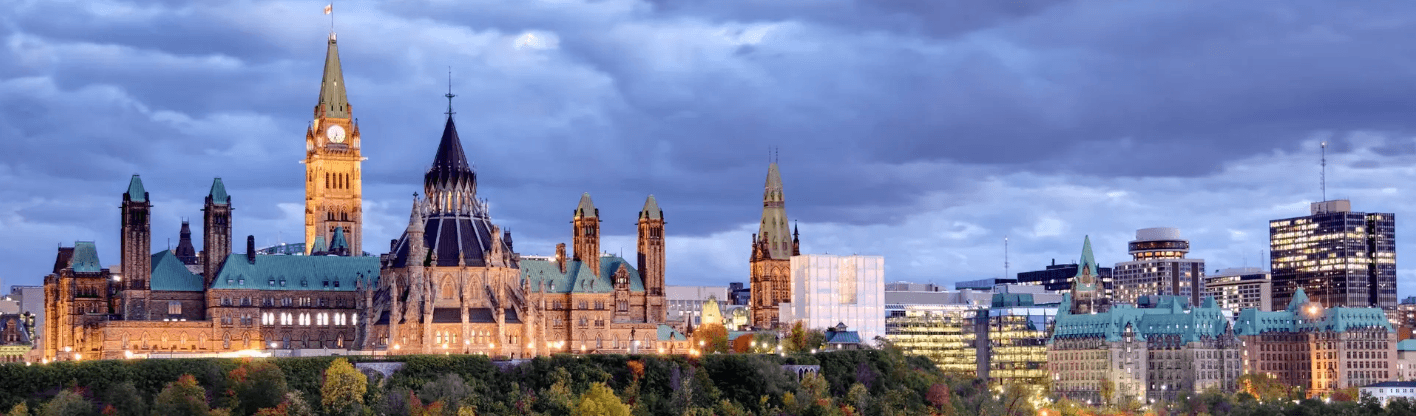
Featured insights
US commercial real estate transaction analysis – Q1 2025
A quarterly review of US commercial real estate transaction activity across all property types.
FEATURED episode
EP67 - CRE’s mid-year market pulse: Rates, risks, and real assets
We share the key macroeconomic signals and policy decisions affecting commercial real estate as we move into the second half of 2025. From declining retail sales and builder sentiment to insights from the latest FOMC meeting, we explore how economic headwinds are influencing CRE strategy, pricing gaps, and transaction volume.
Tune in for analysis on capital access, operational priorities, the evolving definition of real assets, and takeaways from the recent CREFC Annual Conference.
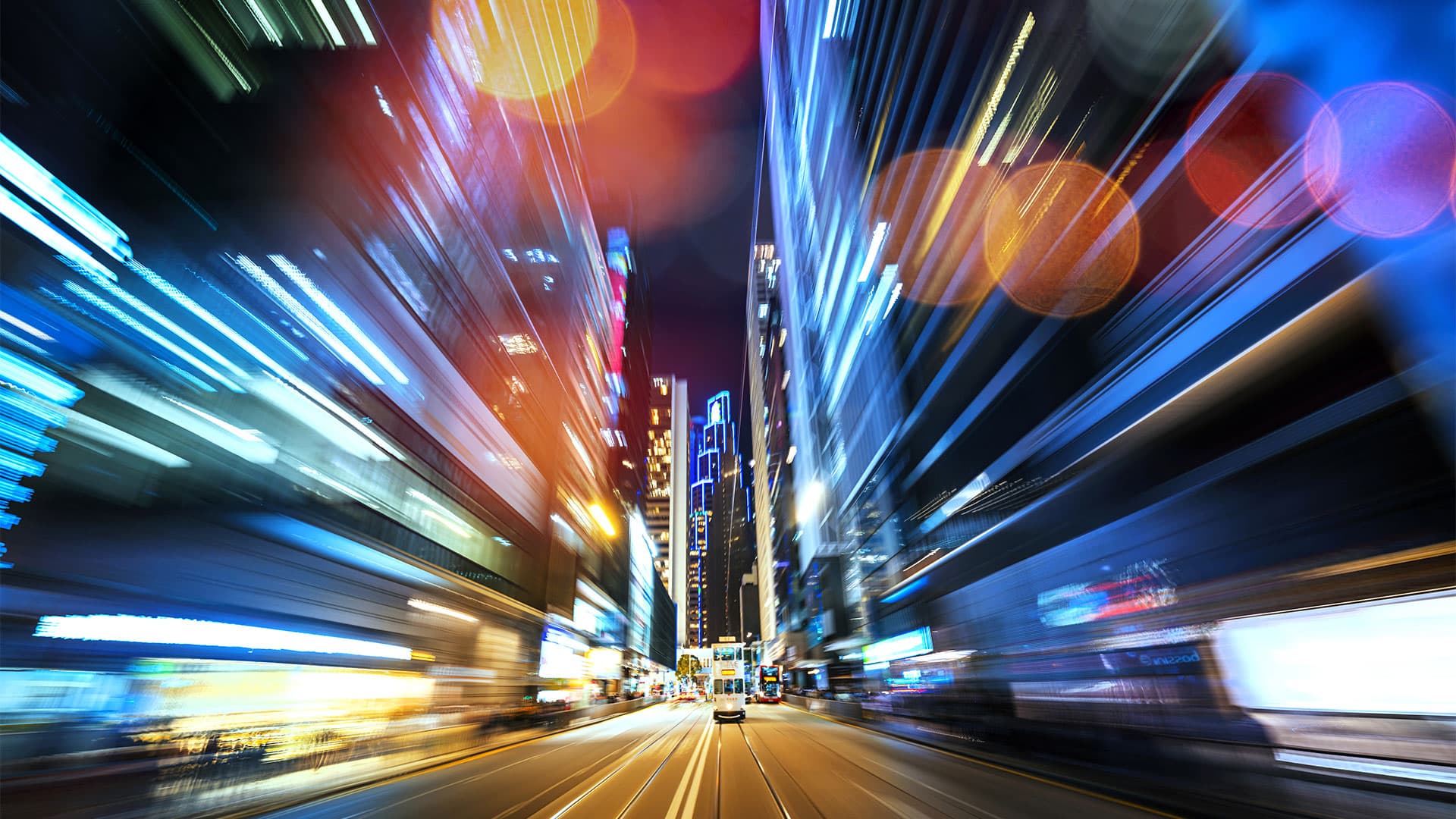